|
|
Mohammad Romel Bhuia, Md Atiqul Islam, Bright I Nwaru, Christopher J Weir, and Aziz Sheikh
Abstract
Background
Statistical models are increasingly being used to estimate and project the prevalence and burden
of asthma. Given substantial variations in these estimates, there is a need to critically assess
the properties of these models and assess their transparency and reproducibility. We aimed to
critically appraise the strengths, limitations and reproducibility of existing models for
estimating and projecting the global, regional and national prevalence and burden of asthma.
Methods
We undertook a systematic review, which involved searching Medline, Embase, World Health
Organization Library and Information Services (WHOLIS) and Web of Science from 1980 to 2017 for
modelling studies. Two reviewers independently assessed the eligibility of studies for inclusion
and then assessed their strengths, limitations and reproducibility using pre-defined quality
criteria. Data were descriptively and narratively synthesised.
Results
We identified 108 eligible studies, which employed a total of 51 models: 42 models were used to
derive national level estimates, two models for regional estimates, four models for global and
regional estimates and three models for global, regional and national estimates. Ten models were
used to estimate the prevalence of asthma, 27 models estimated the burden of asthma –
including, health care service utilisation, disability-adjusted life years, mortality and direct
and indirect costs of asthma – and 14 models estimated both the prevalence and burden of
asthma. Logistic and linear regression models were most widely used for national estimates.
Different versions of the DisMod-MR- Bayesian meta-regression models and Cause Of Death Ensemble
model (CODEm) were predominantly used for global, regional and national estimates. Most models
suffered from a number of methodological limitations – in particular, poor reporting,
insufficient quality and lack of reproducibility.
Conclusions
Whilst global, regional and national estimates of asthma prevalence and burden continue to inform
health policy and investment decisions on asthma, most models used to derive these estimates
lack the required reproducibility. There is a need for better-constructed models for estimating
and projecting the prevalence and disease burden of asthma and a related need for better
reporting of models, and making data and code available to facilitate replication.
|
Resources are limited and health system planners need to make investment decisions based on available
evidence of disease prevalence, associated morbidity and health care utilisation, mortality and costs, how
these are likely to have the potential to change over time and their amenability to interventions [1-8]. Modelling studies have the
potential to inform such important deliberations [1,9-11], hence, they have been
prioritised by inter-governmental organisations such as the World Health Organization (WHO) and funders such
as Bill and Melinda Gates Foundation [3,5,8,12-
17]. The Institute for Health Metrics and Evaluation (IHME) [18] and The Child Health Epidemiology Reference Group (CHERG) [19] are two prominent examples of initiatives that have extensively
used modelling approaches to generate estimates of disease epidemiology and morbidity.
Focusing specifically on estimates of asthma prevalence and burden, we observe that varying estimates have
been reported in the published literature at national, regional and global levels, which are not consistent
over the years (as shown in
Table 1
). Given the range of models used to estimate prevalence and burden of asthma, it is a challenging
task to determine an appropriate model that can be applied in a new data set. Furthermore, there is a lack
of a robust critical evidence base to help guide decisions on which model(s) are most appropriate for
different contexts. There is, therefore, a need for a systematic appraisal of the merits and limitations of
available models. In this study, we sought to systematically describe and critique existing models for
prevalence and burden of asthma in relation to their strengths, limitations and reproducibility.
Table 1.
Global prevalence and burden (mortality) of asthma
Study |
Year |
Prevalence in thousands (uncertainty interval)
|
Burden: mortality in thousands (uncertainty
interval) |
GBD [12,
13]
|
2017
|
272 677 (242 295-304 699)
|
495 (338-641)
|
GBD [3,17]
|
2016
|
339 440 (319 582-360 796)
|
420 (338.8-517.7)
|
GBD [14]
|
2015
|
358 198 (323 134-393 466)
|
397 (363-439)
|
GBD [5,8]
|
2013
|
241 695 (238 151-245 465)
|
489 (397.7-676.8)
|
GBD [1,9]
|
2010
|
334 247 (Not available)
|
345.7 (282.6–529.1)
|
WHO [20]
|
2004
|
234 900 (Not available)
|
287 (Not available)
|
|
|
|
|
METHODS
Protocol
We described and documented the methods employed in this systematic review in detail in a previously
published protocol [21] and therefore confine ourselves here
to a summary of the methods employed.
Eligibility criteria
We included any study that applied models for estimating and projecting prevalence or disease burden
of asthma. We included original research and review articles, including systematic reviews,
meta-analyses and meta-syntheses of observational studies in human populations of any age and sex.
We included research articles from any country and any setting (urban/rural) and published in any
language. Our outcomes of interest were the prevalence of asthma and different components of the
disease burden of asthma. The components of disease burden were direct and indirect costs of asthma
(costs due to primary care utilisation, hospitalisation, ambulatory care, emergency visit, drug
cost, absenteeism, presentism), disability-adjusted life years (DALYs), years lived with disability
(YLDs), years of life lost (YLLs), potential years of life lost, healthy years of life lost, active
life expectancy, disability-free life expectancy, disability-adjusted life expectancy, and healthy
life expectancy (HALE).
Information sources
We identified relevant published and unpublished studies through searching electronic databases,
hand-searching of pertinent journals and checking reference lists of all the eligible papers for
studies published between January 1980 and September 2017. The following electronic databases were
searched: Medline, Embase, World Health Organization Library and Information Services (WHOLIS
library catalogue of books and reports) and Web of Science Core Collection. Journals that were
hand-searched included The Lancet, BMJ (1980-2017), European Respiratory Journal (1988-2017), Lancet
Respiratory Medicine (2013-2017), Lancet Global Health (2013-2017) and Journal of Global Health
(2011-2017). We ran the last searches on 16 September 2017. We included papers published in any
language and translated the papers that were not in English with the help of fluent or native
speakers.
Search strategy
Comprehensive search strategies were developed for all the aforementioned databases in consultation
with a senior medical librarian at The University of Edinburgh to identify both published and
unpublished (grey literature) primary studies as well as reviews. The search terms used included but
were not limited to asthma, wheeze, epidemiology, prevalence, burden, morbidity, mortality, DALY,
QALY, HALE, primary health care, emergency service, hospitalisation, absenteeism, cost of illness,
model, estimate, projection. The detailed search strategies used to search each database are given
in Appendix S1 in the
Online Supplementary Document
.
Study selection
Two reviewers independently checked and screened the titles and abstracts of identified articles
against the inclusion criteria. Full-text copies of potentially eligible studies were obtained and
assessed by two independent reviewers (MRB and MAI) on the basis of the inclusion criteria.
Discussion between the two reviewers resolved the majority of discrepancies; a third reviewer (BIN)
arbitrated in the case of any disagreements.
Data collection process
We developed a data extraction form and used it to extract relevant data from included studies. The
data extraction form was piloted on 10 included studies and was then refined accordingly prior to
full use in the review. Data extraction was performed independently by two reviewers (MRB and MAI).
Any discrepancies in data extraction were resolved through discussion between the reviewers, with
arbitration by a third reviewer (BIN) if a decision could not be reached.
Data items
The following data items were extracted from each paper: 1) study identification (authors’
name, study/publication year, title); 2) aims and methods of the study (context of the study;
country or region; study outcomes; burden type; case definitions; type of estimation; data and study
population: data sources, age, sex, study area; estimation/study period; study design; data type;
response variables; and data level); 3) model information (model name; model purpose; model
structure; appropriateness of the model; model assumptions; model building; model fitting; model
diagnosis; goodness-of-fit; robustness; missing data; uncertainty estimation; validation;
sensitivity analysis; adequate model presentation; adequate reporting of estimates; reproducibility:
availability of input data, computer coding and model fitting manual).
Summary measures
As this review mainly focused on the properties of models rather than quantitative measures, we did
not perform any quantitative synthesis of the data.
Methods of analysis
We produced a tabular summary of the data to summarise the overall evidence. Descriptive statistics
and graphical presentation were used to summarise the results. A detailed critical narrative
synthesis of the models was undertaken regarding their strengths, limitations and reproducibility.
Quality appraisal of the included models
To our knowledge, there is no existing quality appraisal tool to assess the quality of the components
of models for estimating the prevalence and burden of diseases. We therefore developed our own model
evaluation checklist by adapting relevant sections from pertinent critical appraisal checklists [
22,23], reporting
guidelines [24] and other guidelines for good practice in
modelling studies [25-28
]. Prior to finalising this checklist, we consulted three experts (two medical statisticians
and an epidemiologist) in modelling studies.
Assessing strengths, limitations and reproducibility of the included models
Our checklist consists of 13 quality criteria that a model should possess. We present the checklist
by a pyramid in
Figure 1
in terms of the hierarchy of the model quality criteria. Table S1 in the
Online Supplementary Document
provides a description of these criteria. We classified the model quality criteria into two
groups: 1) fundamental or internal aspects of the modelling process; and 2) external aspects. The
fundamental or internal aspects are: model statement, model structure, model appropriateness, model
assumptions, model building and model fitting. The external aspects are: model diagnosis, testing
goodness-of-fit, addressing missing data, model validation, sensitivity analysis, adequate
presentation and reporting of model to ensure reproducibility.
Figure 1.
Checklist for assessing the quality of models. 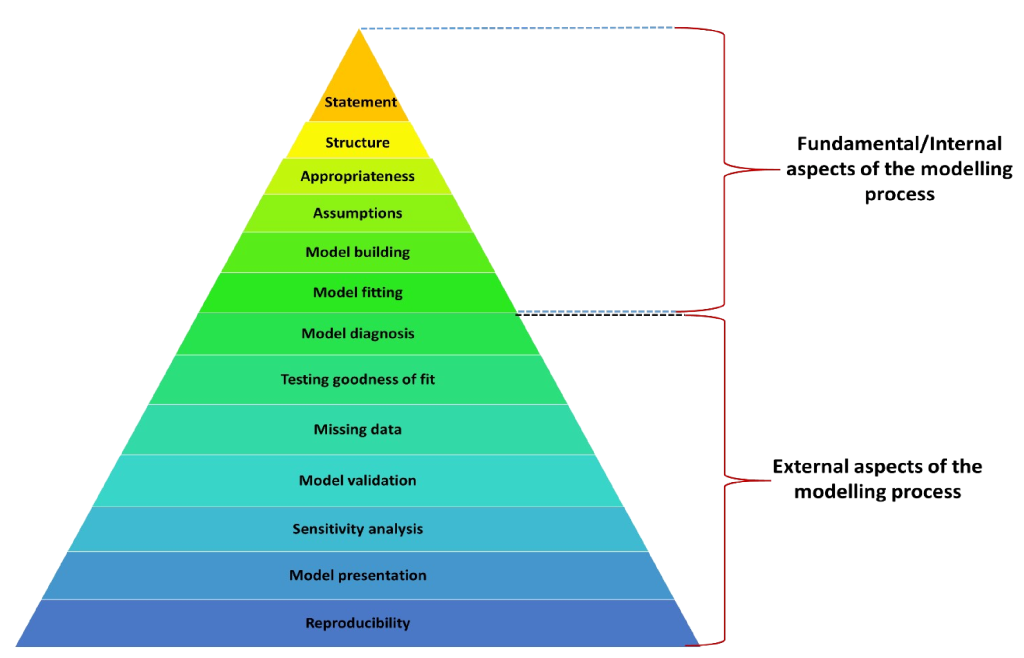
Reporting
This systematic review is reported following the guidelines of the Preferred Reporting Items for
Systematic review and Meta Analysis (PRISMA) checklist [29].
RESULTS
Study selection
Our electronic search of the databases yielded a total of 23 571 references. After removing
duplicates, 18 502 study titles and abstracts were screened against our inclusion criteria.
Among these, 287 full-text articles were assessed in detail. Finally, 92 papers met the inclusion
criteria. An additional 16 papers identified from hand-searching of journals and scrutinising
reference lists of included studies met the inclusion criteria. Therefore, a total of 108 studies
were included in the systematic review. The PRISMA flow diagram of the selected papers is presented
in
Figure 2
. We included five non-English papers: two Dutch papers, one German paper, one French paper
and one Spanish paper.
Figure 2.
PRISMA flow diagram of selected papers. 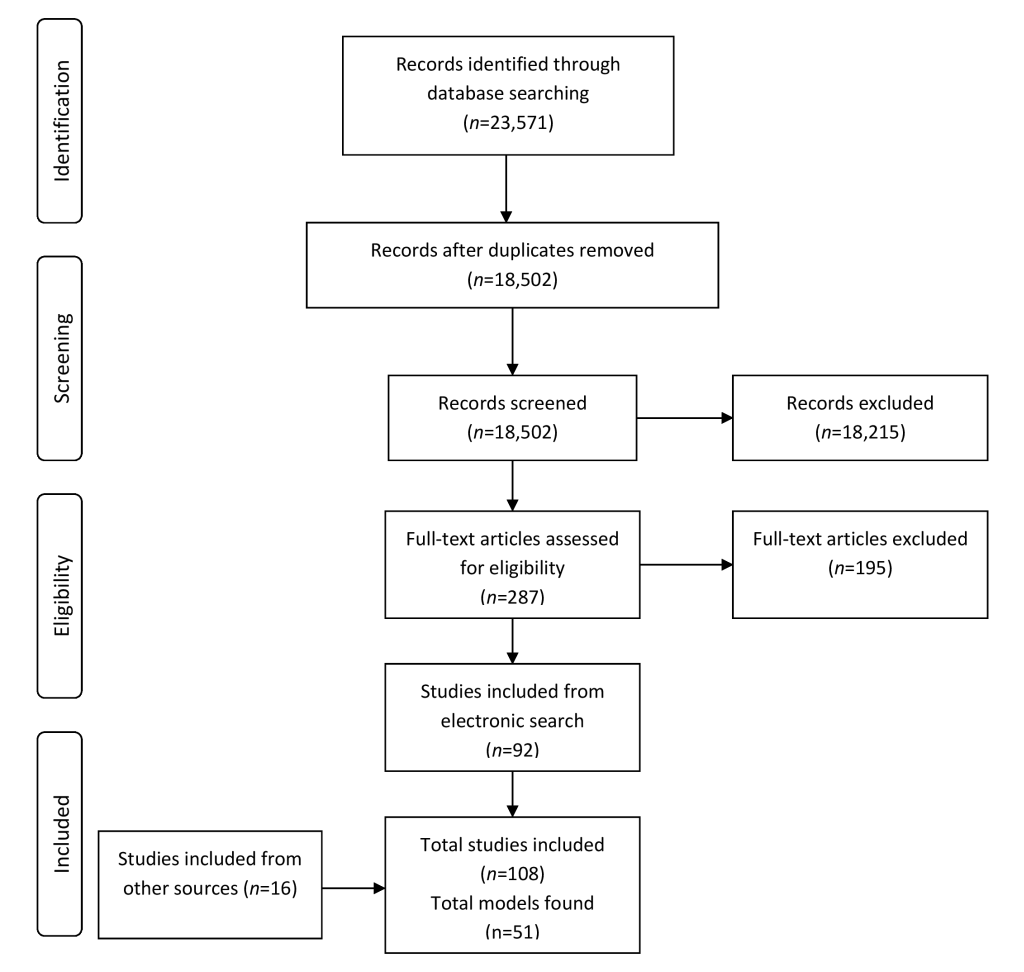
Study characteristics
We identified 94 studies that estimated the prevalence and burden of asthma at a national level; two
at a regional level; seven at both global and regional levels; and five at global, regional and
national levels (
Figure 3
). Among the 108 included studies, 41 studies estimated the prevalence of asthma, 52 studies
estimated the burden of asthma and 15 studies estimated both prevalence and burden of asthma (
Figure 3
). Most of the included studies originated from Europe (n = 33, 30.6%);
followed by North America (n = 29, 26.9%), worldwide or multi-country
(n = 19, 17.6%), Asia (n = 15, 13.9%), South America
(n = 7, 6.5%) and Australia (n = 4, 3.7%); and only one study was from
Africa (
Table 2
).
Figure 3.
Distribution of included studies and models. *One model (linear regression model) was used in
both national-level and global-and-regional-level studies which we counted as national level
model due to its high uses in national level studies; and one model (CODEm) was used in both
global-and-regional-level and global-regional-and national-level studies which we counted as
global-regional-and national-level model due to its high uses in global-regional-and
national-level studies. 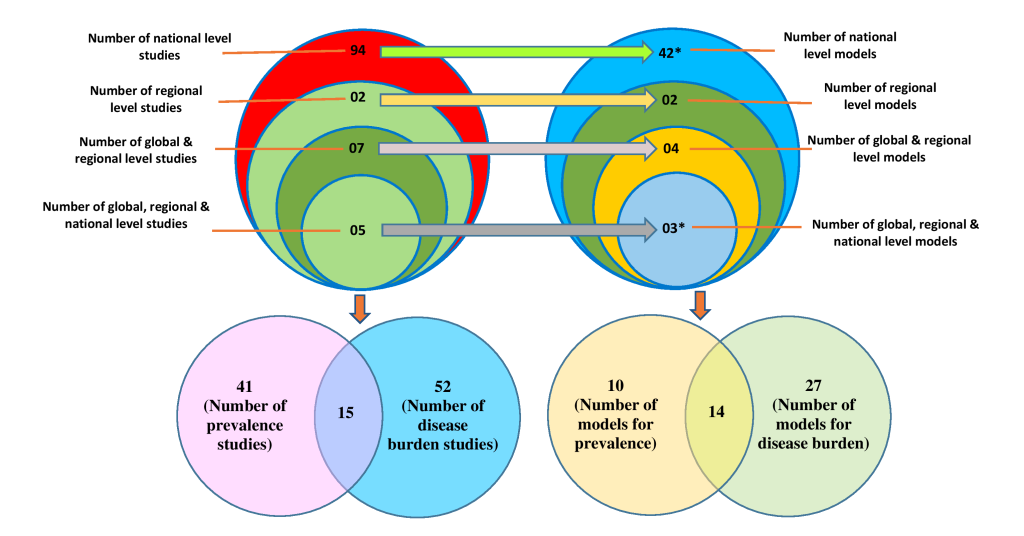 Table 2.
Distribution of included studies by region
Region |
Number of studies (%) |
Africa
|
1 (1.0)
|
Asia
|
15 (13.9)
|
Australia
|
4 (3.7)
|
Europe
|
33 (30.6)
|
North America
|
29 (26.9)
|
South America
|
7 (6.5)
|
Worldwide or multi-country
|
19 (17.6)
|
Total
|
108 (100.0)
|
Models for estimating and projecting prevalence and burden of asthma
A total of 51 models were used in the 108 included studies: 42 models were used to derive national
level estimates (41 national level models plus one model common with global-and-regional level); two
models were used to derive regional level estimates; four were used for global and regional
estimates; and three models were used to derive global, regional and national estimates (
Figure 3
). Among these 51 models, ten models were used to estimate the prevalence of asthma, 27
models were used to estimate the burden of asthma, and 14 models were used to estimate both the
prevalence and burden of asthma (
Figure 3
). Distribution of all the models is presented by study level and type of measurement in
Table 3
.
Table 3.
Distribution of models by study level and type of measurement
Study level
|
Type of measurement
|
Prevalence
|
Burden
|
Both prevalence and burden
|
National
|
1. Meta-analysis: random effect model
|
1. Two-part models
|
1. Logistic regression model
|
2. Logistic regression
model with regression splines/restricted cubic splines
|
2. Generalised linear models with gamma distribution and
logarithmic link function
|
2. Linear regression model*
|
3. Exponential regression model
|
3. Log transformed linear model
|
3. Poisson regression model
|
4. General linear predictive model
|
4. LOESS (locally weighted regression) model
|
4. Negative binomial regression model
|
5. Hierarchical logistic
regressions model
|
5. Bootstrapped prevalence-based cost of illness model
|
5. Generalised estimating equations (GEE)
|
6. Survey weighted logistic
regression model
|
6. Box-Jenkins regression-ARIMA model
|
6. Generalised linear models
|
|
7. Conditional Autoregressive (CAR) model
|
7. Generalised linear mixed effect model
|
|
8. Cost assessment model
|
8. Computer simulation model
|
|
9. Economic model
|
9. Double exponential smoothing model
|
|
10. Exchangeable (EX) Model – Poisson-Gamma Model
|
10. Epidemiological model based on a dynamic multi-state
lifetable
|
|
11. First degree homogeneous Markov model
|
11. RIVM Chronic disease model
|
|
12. Generalised additive model (GAM)
|
|
|
13. Heckman selection model
|
|
|
14. Joinpoint regression model
|
|
|
15. Log-linear autoregression model
|
|
|
16. Log-linear regression model
|
|
|
17. Machine learning based prediction model
|
|
|
18. Multiplicative models for rates (Beslow and Day
method)
|
|
|
19. Multivariate regression model with weighted least
squares
|
|
|
20. Polynomial regression model
|
|
|
21. Quadratic regression model
|
|
|
22. Quantile regression model
|
|
|
23. Seasonal autoregressive integrated moving average
(SARIMA) model
|
|
|
24. Weighted linear regression model
|
|
|
25. Zero-inflated negative binomial regression model
|
|
Regional
|
1. Nonlinear exponential regression model
|
-
|
-
|
2. Meta-analysis: random effects
Bayesian model
|
Global and regional
|
1. DisMod-MR
|
1. Cause Of Death Ensemble modeling (CODEm)†
|
1. DisMod
|
|
2. Cause-of-death modeling (CodMod)
|
2. DisMod II
|
|
3. Linear regression model*
|
|
Global, regional and national
|
1. DisMod-MR 2.0 |
1. Cause Of Death Ensemble modeling (CODEm)† |
1. DisMod-MR 2.1 |
Outcome measures and statistical methods used by the included studies
A tabular summary of the data is presented in Table S2 in the
Online Supplementary Document
to summarise the overall evidence. The included studies mainly used models for deriving
annual estimates, trends, changes of estimates over a period, projections, predicted estimates and
forecasted estimates of prevalence and various components of the disease burden of asthma. In
addition to estimates of prevalence, the components of disease burden that were estimated were
mortality; health care service utilisation (health care provider visit, family practitioner visits,
specialist visits, emergency room visits/emergency department visits, hospitalisation/hospital
admission, re-admission); productivity loss (due to absenteeism, presenteeism and overall work
impairment); cost of asthma – direct medical expenditure (costs of physician office visits,
emergency department visits, outpatient visits, inpatient visits, medications, and other medical
visits, asthma exacerbations and readmissions), indirect costs (costs of absenteeism, costs of
parents’ productivity loss and work-time loss, children’s loss of lifetime earnings
because of premature death), societal costs; incidence; asthma exacerbation; YLDs; and quality of
life.
The frequencies of uses of each model by type of study are shown in
Figure 4
. Logistic regression modelling was the most commonly used approach for estimating the
national prevalence of asthma, which was used in 21 studies [30
-50] (out of 46 studies that estimated national
prevalence). This was followed by linear regression, which was used in 14 studies [51-64] (out of 57
studies) to estimate and project the national burden of asthma. DisMod, DisMod II and cause of death
modelling (CodMod) were used to estimate global and regional prevalence and burden of asthma in four
studies [10,20,65,66] (out of seven
studies). Different versions of DisMod-MR-Bayesian meta-regression models (DisMod-MR, DisMod-MR 2.0
and DisMod-MR 2.1) and Cause Of Death Ensemble modelling (CODEm - mixed effects linear/nonlinear
models and/or spatial-temporal Gaussian process regression models) were used in all the five studies
[3,5,8,14,17] for estimating the global, regional and national prevalence
and burden of asthma.
Figure 4.
Frequencies of uses of each model by type of study. NB. Sum of the frequencies of uses of a
model may not be equal to the number of studies used that model, because many studies used
more than one model and some studies used same model for estimating both prevalence and more
than one component of burden. 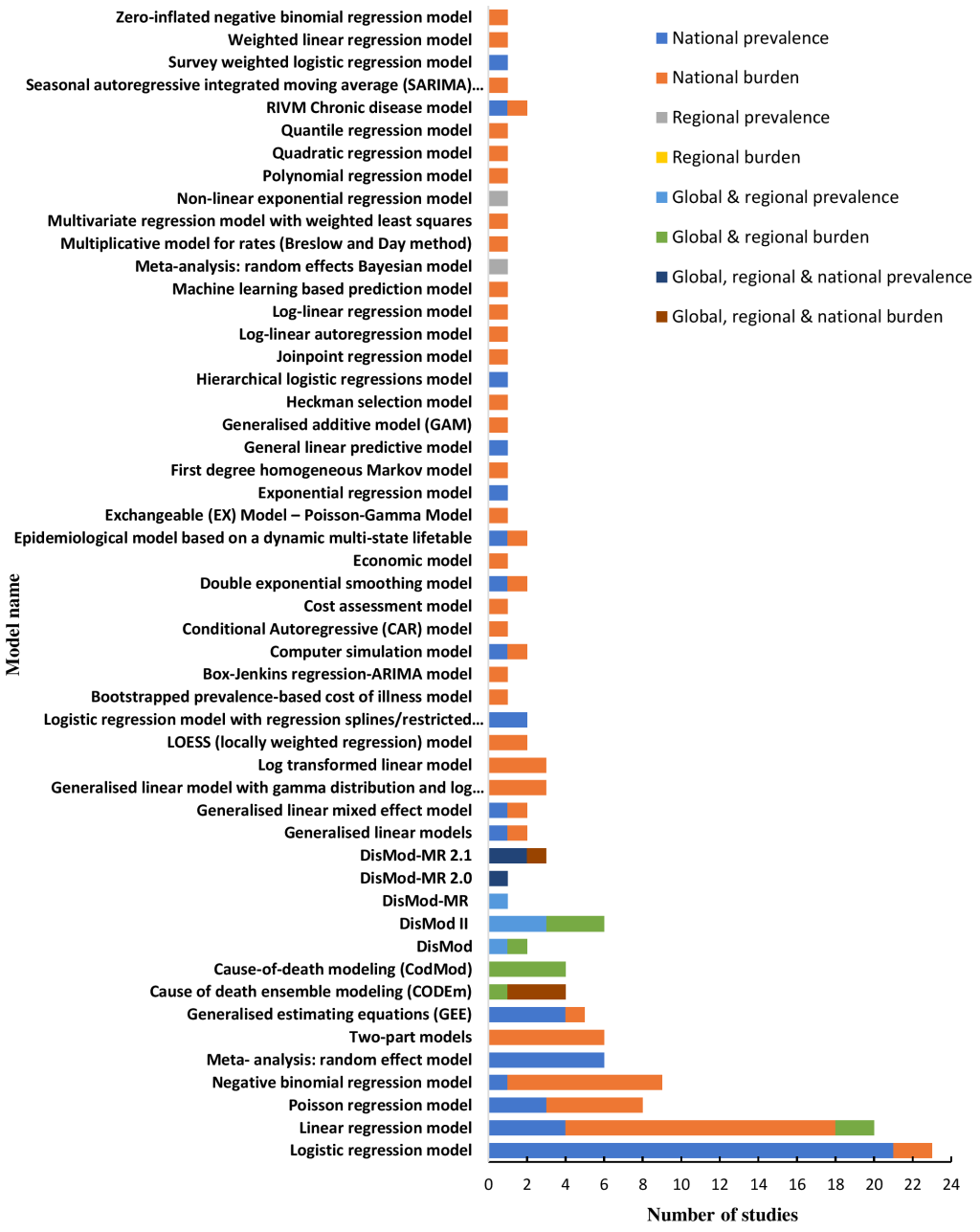
Strengths, limitations and reproducibility of the included models
Figure 5
presents the results emanating from the application of the checklist we developed to
appraise the quality of models.
Figure 5.
Percentage of studies fulfilled each model quality criteria. 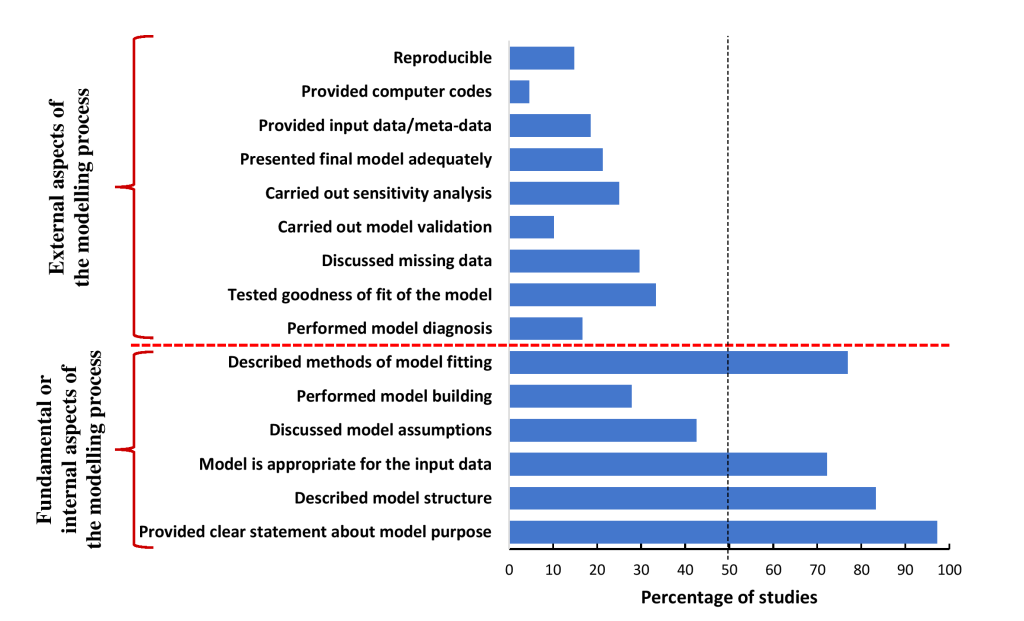 Strengths of the models
More than half of the studies fulfilled four of the six internal model quality criteria. One
hundred and five (97.2%) studies [1,3,5,8
-10,14,
17,20,30,32,33,35-127] provided a clear statement about the questions that
the models aimed to answer. Four in five studies (n = 90; 83.3%) [1,3,5,8,10,14,17,20,30,33,34,36-44,46-51,53-55,57,59-70,72,73,75-78,81-84,87,89-111,113-120,122-127] explicitly described the structure of the models, and
nearly three-quarters of studies (n = 78; 72.2%) [1
,3,5,8-10,14,17,20,30,33-44,46-50,65-67,70-72,75-77,81,82,84,85,87-94,96-114,116-120,123-128] used
appropriate modelling tools to deal with the nature, distribution and type of input data.
Adequate description of the process of model fitting was provided by 83 (76.9%) studies [1,3,5,8-10,14,17,20,34-44,46-51,53-55,57,60-66,68-70,72,76,78,81-84,87,89-111,113-119,122,124-127] (
Figure 5
).
Limitations of the models
Most studies failed to fulfil two internal model quality criteria and all the external criteria.
Sixty-two (57.4%) studies [30,32-34,36-38,40,41,44-59,61-64,67,68,71,73,74,79,81,83,85,87,88,92,93,95,96,98-101,104,106,109-115,117,122,127-129] did not provide any information about the model assumptions, while,
only 30 (27.8%) studies [1,
3,8,14,
33,36,38,44,50,55,57,67,70,72,75-77,82,84,90-92,94,96,97,100,103,110,116,124] performed
model building to select necessary variables for the models. The model diagnosis (model adequacy
checking) was performed by 18 (16.7%) studies [49,68,69,72,75,76,82,86,89-91,93,97,101,103,105,107,118], whereas
36 (33.3%) studies [1,3
,5,8,14,46,49-51,54-56,60,61,63,64,67,69,72,81,82,84,89-91,94,96,97,103,107,110,117-119,122,124] tested
and reported goodness-of-fit of the models. Very few studies discussed missing data
(n = 32; 29.6%) [1,3,5,8
-10,14,
17,20,32,33,44,46,48,58,65,66,70,76,78,85,86,89,92,94,97,109,114,117,118,124,126], carried out model validation (n = 11;
10.2%) [1,3,5,8,14,41,80,84,96,97,123], performed sensitivity analysis
(n = 27; 25%) [1,10,14,20,41,43,45,55,65,72,75,76,78-80,84,88,93,104,108,112,118-121,123,124] and presented final model adequately (n = 23; 21.3%) [
40,51,53-56,62,69,75,81,84,89,90,93-95,97,99,101,106,107,117,124] (
Figure 5
).
Reproducibility
We found only 20 (18.5%) studies [3,14,17,49
,51,52,54,62,69,81,90,93,98,99,101,104,110,114,117,122] that provided
final input data or meta-data. Very few studies (n = 5; 4.6%) [3,14,17
,90,117]
provided the computer code used to fit the models, and very few models (n = 16; 14.8%)
[49,51,54,62,69,81,90,93,98,99,101,104,110,114,117,122] were
therefore judged to be reproducible (
Figure 5
).
Further analysis, assessing whether the included studies adhered the new Guidelines for Accurate and
Transparent Health Estimates Reporting (GATHER) [24] showed
that most of the included studies (n = 105; 97%) did not adhered the GATHER guidelines
to report their derived estimates of prevalence and burden of asthma; nevertheless, 82%
(n = 88) of these studies were published before the publication of GATHER guidelines.
Among the 20 studies, that were published after the availability of GATHER guidelines, only three
studies (15%) [3,14,
18] adhered these guidelines to report their estimates.
DISCUSSION
Statement of principal findings
This systematic review of the international literature has found that a variety of models are used to
estimate disease prevalence and burden; most models, however, suffer from methodological
limitations, in particular, lack of reproducibility and sub-optimal reporting.
Almost all the studies provided a clear statement about the questions that the models aimed to
answer. The majority of the studies described the structure of the models explicitly; mentioned the
methods used to fit the models or used to estimate model parameters; and applied appropriate
modelling tools to deal with the nature, distribution and type of input data. These were the
strengths of the models. However, these models had substantial limitations. We observed a lack of
clarity in reporting the models. The majority of the studies did not provide any information on the
model assumptions, methods of model building, methods of model diagnosis, methods of handling
missing data, model validation and sensitivity analysis. In addition, many studies did not
adequately present the final model parameter estimates, standard errors and confidence intervals.
Moreover, most of the models could not be reproduced as the studies did not provide input data (or
alternatively meta-data, synthetic data or simulated data if input data could not be published due
to confidentiality), computer coding, a model fitting manual or complete information about the
process of model formulation.
Strengths and limitations
The main strengths of this review include the comprehensive search strategy employed, the use of
established methodology (two independent reviewers for screening, full-text assessment and data
extraction) and the inclusion of studies from all over the world including studies published in any
language. Critical appraisal of identified models and expert involvement in developing model
assessment tools further strengthened the credibility of this review. However, this review has a
number of potential limitations. As with any systematic review, we may have missed some studies.
Although risk factor based models or association models are particularly essential to assess
determinants of a disease, we did not include studies that used models to estimate risk factors or
to assess association rather than estimating prevalence and disease burden using model. The use of a
self-developed customised model evaluation tools, due to the lack of appropriate critical appraisal
checklist, may be considered to be a further limitation of this review. There is a need for the
development of an internationally standard tool that will be used for the purpose.
Interpretation in the context of the wider literature
This systematic review is the first to synthesise models for prevalence and burden of asthma in the
context of national, regional and global estimates and projections. Previous systematic reviews on
disease modelling studies were mainly undertaken on other domains, such as prediction models [130-139], economic
models [140] and decision analytic models [141-143]. Most of the
findings of our review are in line with these previous reviews, suggesting that inadequate model
development and poor reporting quality are the key issues in modelling studies that chiefly affect
the quality of model-derived estimates and hinder the assessment of the usability of the models. A
systematic review on projection models for prevalence and burden of chronic obstructive pulmonary
disease (COPD) [144] argued that there was no consensus on
the best model structure as models varied depending on the purpose and contexts of modelling.
Another review on coronary heart disease policy models [25]
emphasised introducing standard reporting guidelines to improve the reporting quality of models.
Implications for policy, practice and future research
Implication for asthma policy
Existing estimates are heavily reliant on modelling studies due to lack of data on direct
measurements of asthma cases in many countries and regions. Whilst these modelling studies have
advanced better understanding and appreciation of the burden of different diseases, the lack of
reproducibility of the models, as highlighted in this review, requires concerted effort from
researchers and decision makers to set in place platforms that will ensure that estimates of
disease burden produced can be reproduced. Policymakers should thus be aware of the transparency
of modelling processes and the reliability of the input data when making decisions on the basis
of these model-based estimates.
Implications for model developers
The findings of this review suggest that models should be carefully designed to incorporate all
the necessary methodological components required to develop a robust model, including an
explicit statement about the purpose and structure of the model; statement of necessary model
assumptions; variable selection applying appropriate techniques; model diagnostic accuracy
checking; assessing goodness-of-fit; addressing missing data by applying suitable techniques;
applying optimum methods of parameter estimation; carrying out sensitivity analysis; and
performing both internal and external model validation. Besides, a highly complex model usually
lacks understanding, usability, reproducibility and, hence, credibility. While publishing
models, sufficient information about the complete modelling process, therefore, should be
reported to facilitate its understanding and usability for non-technical audiences. For example,
a model development manual should be made publicly available, including input data and necessary
computer code, to describe the step-by-step process of model development with illustrative
examples. Although the perspectives of this review are prevalence and disease burden of asthma,
these recommendations also apply to the modelling prevalence and burden of other chronic
diseases.
Implication for future research
Future research could potentially be undertaken to develop consensus guidelines for developing or
fitting and reporting models for prevalence and burden of diseases. Moreover, developing a
critical appraisal checklist for assessing the quality of models for prevalence and burden of
diseases is another key area for future research.
Of the available approaches, we found the Bayesian meta-regression method- DisMod-MR, DisMod-MR
2.0, DisMod-MR 2.1 and CODEm [1,3,5,8
,9,14,17] modelling tools faired best as they fulfilled most of
our model quality criteria and were specially designed to deal with the diversity of data
(multiple sources and designs) [11,145] needed to derive national, regional and global, level estimates.
However, these modelling methods lack usability for the general user because of unavailability
of sufficient technical detail and customised packages in standard statistical software such as
R, SAS, and STATA. Therefore, more work needs to be done with these models to improve their
usability. Moreover, DisMod-MR and CODEm are used as generic models by the Global Burden of
Disease (GBD) collaborators to derive health estimates for numerous diseases and injuries.
Therefore, the potential added value of well-constructed asthma-specific models should be
considered.
Conclusions
Amidst data types and their sources, modelling remains indispensable for estimating the prevalence and
burden of disease. This evidence synthesis has shown that existing models that have been applied to
estimate the prevalence and burden of asthma suffer from methodological limitations, in particular,
suboptimal reporting and lack of reproducibility. There is a need to enhance the reportage of models
used for estimating and projecting the prevalence and disease burden of asthma and making data and code
available to facilitate replication. Moreover, there is also a need for developing better-constructed
asthma-specific models in an attempt to produce more accurate and consistent estimates. In the interim,
we suggest using Bayesian meta-regression models and cause of death ensemble models for estimating
national, regional and global prevalence and burden of asthma, and Box-Jenkins regression-
autoregressive integrated moving average (ARIMA) model to make projections in relation to these
estimates. We also suggest to validate the Bayesian meta-regression models against their alternative
frequentist or classical models to check which modelling approaches generate better estimates of
prevalence and burden of asthma than the others.
Acknowledgements
We are grateful to Professor Jackie Price, Professor Steff Lewis and Dr Niall Anderson for their support
as experts to develop the checklist for assessing the quality of the models included in our review. We
are also grateful to Marshall Dozier, Senior Liaison Librarian for the College of Medicine and
Veterinary Medicine, The University of Edinburgh, for her support in developing the search strategies.
Finally, we express our gratitude to Fuentes Pacheco Andrea Carolina, Cameron Werner, Dewi Peerlings and
Sumonkanti Das for helping with the translation of papers to English from other languages.
REFERENCES
[1]
R Lozano, M Naghavi, K Foreman, S Lim, K Shibuya, and V Aboyans. Global and regional mortality from 235 causes of death for 20 age groups in 1990 and
2010: a systematic analysis for the Global Burden of Disease Study 2010. Lancet. 2012;380:2095-128
. DOI: 10.1016/S0140-6736(12)61728-0. [PMID:23245604]
[2]
RM Staruch, A Beverly, JK Sarfo-Annin, and S Rowbotham. Calling for the next WHO Global Health Initiative: the use of disruptive innovation
to meet the health care needs of displaced populations. J Glob Health. 2018;8:010303
. DOI: 10.7189/jogh.08.010303. [PMID:29977527]
[3]
. Global, regional, and national age-sex specific mortality for 264 causes of death,
1980-2016: a systematic analysis for the Global Burden of Disease Study 2016. Lancet. 2017;390:1151-210
. DOI: 10.1016/S0140-6736(17)32152-9. [PMID:28919116]
[4]
S Schütte, PNM Acevedo, and A Flahault. Health systems around the world - a comparison of existing health system rankings.
J Glob Health. 2018;8:010407
. DOI: 10.7189/jogh.08.010407. [PMID:29564084]
[5]
. Global, regional, and national incidence, prevalence, and years lived with disability
for 301 acute and chronic diseases and injuries in 188 countries, 1990-2013: a systematic
analysis for the Global Burden of Disease Study 2013. Lancet. 2015;386:743-800
. DOI: 10.1016/S0140-6736(15)60692-4. [PMID:26063472]
[6]
I Rudan, S Yoshida, KY Chan, S Cousens, D Sridhar, and R Bahl. Setting health research priorities using the CHNRI method: I. Involving funders.
J Glob Health. 2016;6:010301
. DOI: 10.7189/jogh.06.010301. [PMID:26401269]
[7]
S Zhang, B Incardona, SA Qazi, K Stenberg, H Campbell, and H Nair. Cost-effectiveness analysis of revised WHO guidelines for management of childhood
pneumonia in 74 Countdown countries. J Glob Health. 2017;7:010409
. DOI: 10.7189/jogh.07.010409. [PMID:28400955]
[8]
. Global, regional, and national age-sex specific all-cause and cause-specific
mortality for 240 causes of death, 1990-2013: a systematic analysis for the Global Burden of
Disease Study 2013. Lancet. 2015;385:117-71. DOI: 10.1016/S0140-6736(14)61682-2. [PMID:25530442]
[9]
T Vos, AD Flaxman, M Naghavi, R Lozano, C Michaud, and M Ezzati. Years lived with disability (YLDs) for 1160 sequelae of 289 diseases and injuries
1990-2010: a systematic analysis for the Global Burden of Disease Study 2010. Lancet. 2012;380:2163-96
. DOI: 10.1016/S0140-6736(12)61729-2. [PMID:23245607]
[10]
Mathers CD, Lopez AD, Murray CJL. The Burden of Disease and
Mortality by Condition: Data, Methods, and Results for 2001. In: Lopez AD, Mathers CD, Ezzati M,
Jamison DT, Murray CJL, editors. Global Burden of Disease and Risk Factors. Washington (DC): The
World Bank; 2006. p. 45-93.
[11]
KJ Foreman, R Lozano, AD Lopez, and CJ Murray. Modeling causes of death: an integrated approach using CODEm. Popul Health Metr. 2012;10:1 DOI: 10.1186/1478-7954-10-1. [PMID:22226226]
[12]
. Global, regional, and national incidence, prevalence, and years lived with disability
for 354 diseases and injuries for 195 countries and territories, 1990–2017: a systematic
analysis for the Global Burden of Disease Study 2017. Lancet. 2018;392:1789-858
. DOI: 10.1016/S0140-6736(18)32279-7. [PMID:30496104]
[13]
. Global, regional, and national age-sex-specific mortality and life expectancy,
1950–2017: a systematic analysis for the Global Burden of Disease Study 2017.
Lancet. 2018;392:1684-735
. DOI: 10.1016/S0140-6736(18)31891-9. [PMID:30496102]
[14]
. Global, regional, and national deaths, prevalence, disability-adjusted life years,
and years lived with disability for chronic obstructive pulmonary disease and asthma, 1990-2015:
a systematic analysis for the Global Burden of Disease Study 2015. Lancet Respir Med. 2017;5:691-
706. DOI: 10.1016/S2213-2600(17)30293-X. [PMID:28822787]
[15]
L Liu, S Oza, D Hogan, J Perin, I Rudan, and JE Lawn. Global, regional, and national causes of child mortality in 2000-13, with projections
to inform post-2015 priorities: an updated systematic analysis. Lancet. 2015;385:430-40. DOI: 10.1016/S0140-6736(14)61698-6. [PMID:25280870]
[16]
CF Lanata, CL Fischer-Walker, AC Olascoaga, CX Torres, MJ Aryee, and RE Black. Global causes of diarrheal disease mortality in children <5 years of age: a
systematic review. PLoS One. 2013;8:e72788. DOI: 10.1371/journal.pone.0072788. [PMID:24023773]
[17]
. Global, regional, and national incidence, prevalence, and years lived with disability
for 328 diseases and injuries for 195 countries, 1990-2016: a systematic analysis for the Global
Burden of Disease Study 2016. Lancet. 2017;390:1211-59
. DOI: 10.1016/S0140-6736(17)32154-2. [PMID:28919117]
[20]
World Health Organization. The global burden of disease: 2004
update. Geneva: World Health Organization; 2008.
[21]
MR Bhuia, BI Nwaru, CJ Weir, and A Sheikh. Models for estimating and projecting global, regional and national prevalence and
disease burden of asthma: Protocol for a systematic review. BMJ Open. 2017;7:e015441. DOI: 10.1136/bmjopen-2016-015441. [PMID:28515197]
[22]
KG Moons, JA de Groot, W Bouwmeester, Y Vergouwe, S Mallett, and DG Altman. Critical appraisal and data extraction for systematic reviews of prediction modelling
studies: the CHARMS checklist. PLoS Med. 2014;11:e1001744
. DOI: 10.1371/journal.pmed.1001744. [PMID:25314315]
[24]
GA Stevens, L Alkema, RE Black, JT Boerma, GS Collins, and M Ezzati. Guidelines for Accurate and Transparent Health Estimates Reporting: the GATHER
statement. PLoS Med. 2016;13:e1002056
. DOI: 10.1371/journal.pmed.1002056. [PMID:27351744]
[25]
B Unal, S Capewell, and JA Critchley. Coronary heart disease policy models: a systematic review. BMC Public Health. 2006;6:213 DOI: 10.1186/1471-2458-6-213. [PMID:16919155]
[26]
MC Weinstein, B O’Brien, J Hornberger, J Jackson, M Johannesson, and C McCabe. Principles of good practice for decision analytic modeling in health-care evaluation:
report of the ISPOR Task Force on Good Research Practices–Modeling Studies.
Value Health. 2003;6:9-17
. DOI: 10.1046/j.1524-4733.2003.00234.x. [PMID:12535234]
[27]
F Kirsch. Economic Evaluations of Multicomponent Disease Management Programs with Markov
Models: A Systematic Review. Value Health. 2016;19:1039-54
. DOI: 10.1016/j.jval.2016.07.004. [PMID:27987631]
[28]
MC Weinstein, EL Toy, EA Sandberg, PJ Neumann, JS Evans, and KM Kuntz. Modeling for health care and other policy decisions: uses, roles, and validity.
Value Health. 2001;4:348-61
. DOI: 10.1046/j.1524-4733.2001.45061.x. [PMID:11705125]
[29]
D Moher, A Liberati, J Tetzlaff, and DG Altman. Preferred reporting items for systematic reviews and meta-analyses: the PRISMA
statement. PLoS Med. 2009;6:e1000097
. DOI: 10.1371/journal.pmed.1000097. [PMID:19621072]
[30]
S Bardal, A Smith, HA Luo, T Zhang, G Groeneweg, and R Jimenez Mendez. Asthma in British Columbia: Are we finally breathing easier? A population-based study
of the burden of disease over 14 years. J Asthma. 2017;54:308-17
. DOI: 10.1080/02770903.2016.1208223. [PMID:27414432]
[31]
AL James, MW Knuiman, ML Divitini, J Hui, M Hunter, and LJ Palmer. Changes in the prevalence of asthma in adults since 1966: the Busseltion health
study. Eur Respir J. 2010;35:273-8
. DOI: 10.1183/09031936.00194308. [PMID:19643935]
[32]
G Malik, N Tagiyeva, L Aucott, G McNeill, and SW Turner. Changing trends in asthma in 9-12 year olds between 1964 and 2009. Arch Dis Child. 2011;96:227-31
. DOI: 10.1136/adc.2010.189175. [PMID:21068081]
[33]
LJ Akinbami, AE Simon, and LM Rossen. Changing Trends in Asthma Prevalence Among Children. Pediatrics. 2016;137:e20152354
. DOI: 10.1542/peds.2015-2354. [PMID:26712860]
[34]
L Kausel, A Boneberger, M Calvo, and K Radon. Childhood Asthma and Allergies in Urban, Semiurban, and Rural Residential Sectors in
Chile. ScientificWorldJournal. 2013;2013:
937935. DOI: 10.1155/2013/937935. [PMID:23766726]
[35]
PGJ Burney, S Chinn, and RJ Rona. Has the prevalence of asthma increased in children? Evidence from the national study
of health and growth 1973-86. BMJ. 1990;300:1306-10. DOI: 10.1136/bmj.300.6735.1306. [PMID:2369661]
[36]
G Brozek, J Lawson, D Szumilas, and J Zejda. Increasing prevalence of asthma, respiratory symptoms, and allergic diseases: Four
repeated surveys from 1993-2014. Respir Med. 2015;109:982-90
. DOI: 10.1016/j.rmed.2015.05.010. [PMID:26153339]
[37]
A Venn, S Lewis, M Cooper, J Hill, and J Britton. Increasing prevalence of wheeze and asthma in Nottingham primary schoolchildren
1988-1995. Eur Respir J. 1998;11:1324-8
. DOI: 10.1183/09031936.98.11061324. [PMID:9657574]
[38]
G Verlato, A Corsico, S Villani, I Cerveri, E Migliore, and S Accordini. Is the prevalence of adult asthma and allergic rhinitis still increasing? Results of
an Italian study. J Allergy Clin Immunol. 2003;111:1232-8. DOI: 10.1067/mai.2003.1484. [PMID:12789222]
[39]
GF Miller, E Coffield, Z Leroy, and R Wallin. Prevalence and Costs of Five Chronic Conditions in Children. J Sch Nurs. 2016;32:357-64
. DOI: 10.1177/1059840516641190. [PMID:27044668]
[40]
A López-Silvarrey-Varela, S Pertega-Diaz, S Rueda-Esteban, JM Sanchez-Lastres, MA San-Jose-Gonzalez, and M Sampedro-Campos. Prevalence and geographic variations in asthma symptoms in children and adolescents
in Galicia (Spain). Arch Bronconeumol. 2011;47:274-
82. DOI: 10.1016/j.arbr.2011.01.004. [PMID:21440354]
[41]
A Linneberg, NH Nielsen, F Madsen, L Frolund, A Dirksen, and T Jorgensen. Secular trends of allergic asthma in Danish adults. The Copenhagen Allergy Study.
Respir Med. 2001;95:258-64
. DOI: 10.1053/rmed.2001.1031. [PMID:11316107]
[42]
T Nurmagambetov, O Khavjou, L Murphy, and D Orenstein. State-level medical and absenteeism cost of asthma in the United States.
J Asthma. 2017;54:357-70
. DOI: 10.1080/02770903.2016.1218013. [PMID:27715355]
[43]
O Kolokotroni, N Middleton, N Nicolaou, S Pipis, KN Priftis, and DK Milton. Temporal changes in the prevalence of childhood asthma and allergies in urban and
rural areas of Cyprus: results from two cross sectional studies. BMC Public Health. 2011;11:858 DOI: 10.1186/1471-2458-11-858. [PMID:22074450]
[44]
X Zhang, T Morrison-Carpenter, JB Holt, and DB Callahan. Trends in adult current asthma prevalence and contributing risk factors in the United
States by state: 2000-2009. BMC Public Health. 2013;13:1156 DOI: 10.1186/1471-2458-13-1156. [PMID:24325173]
[45]
R de Marco, V Cappa, S Accordini, M Rava, L Antonicelli, and O Bortolami. Trends in the prevalence of asthma and allergic rhinitis in Italy between 1991 and
2010. Eur Respir J. 2012;39:883-92
. DOI: 10.1183/09031936.00061611. [PMID:22005911]
[46]
JL Kim, J Brisman, MA Aberg, HB Forslund, A Winkvist, and K Toren. Trends in the prevalence of asthma, rhinitis, and eczema in 15 year old adolescents
over an 8 year period. Respir Med. 2014;108:701-8
. DOI: 10.1016/j.rmed.2014.02.011. [PMID:24703830]
[47]
EP Uphoff, PK Bird, JM Anto, M Basterrechea, A von Berg, and A Bergstrom. Variations in the prevalence of childhood asthma and wheeze in MeDALL cohorts in
Europe. ERJ Open Res. 2017;3:00150-2016
. DOI: 10.1183/23120541.00150-2016. [PMID:28845428]
[48]
NA El-Sharif, B Nemery, F Barghuthy, S Mortaja, R Qasrawi, and Z Abdeen. Geographical variations of asthma and asthma symptoms among schoolchildren aged 5 to
8 years and 12 to 15 years in Palestine: the International Study of Asthma and Allergies in
Childhood (ISAAC). Ann Allergy Asthma Immunol. 2003;90:63-71. DOI: 10.1016/S1081-1206(10)63616-2. [PMID:12546340]
[49]
DK Luyt, PR Burton, and H Simpson. Epidemiological study of wheeze, doctor diagnosed asthma, and cough in preschool
children in Leicestershire. BMJ. 1993;306:1386-90. DOI: 10.1136/bmj.306.6889.1386. [PMID:8518607]
[51]
SOM Prietsch, L Zhang, AR Catharino, L Vauchinski, and FE Rodrigues. Asthma mortality among Brazilian children up to 19 years old between 1980 and 2007.
J Pediatr (Rio J). 2012;88:384-
8. DOI: 10.2223/JPED.2215. [PMID:22851537]
[53]
DP Pesut, MV Bulajic, LM Nagomi-Obradovic, AD Grgurevic, ZB Gledovic, and DR Ponomarev. Asthma mortality in Serbia: A 30-year analysis. Respir Med. 2011;105:S50-3
. DOI: 10.1016/S0954-6111(11)70011-7. [PMID:22015087]
[54]
G Chatkin, JM Chatkin, CC Fritscher, D Cavalet-Blanco, HR Bittencourt, and MR Sears. Asthma mortality in southern Brazil: Is there a changing trend? J Asthma. 2007;44:133-6. DOI: 10.1080/02770900601182483. [PMID:17454328]
[55]
NX Thanh, A Ohinmaa, and C Yan. Asthma-related productivity losses in Alberta, Canada. J Asthma Allergy. 2009;2:43-8
. DOI: 10.2147/JAA.S5072. [PMID:21437143]
[56]
JB van Woensel, WM van Aalderen, MC Kneyber, ML Heijnen, and JL Kimpen. Bronchiolitis hospitalisations in the Netherlands from 1991 to 1999. Arch Dis Child. 2002;86:370-1
. DOI: 10.1136/adc.86.5.370. [PMID:11970935]
[57]
S Tual, P Godard, J Bousquet, and I Annesi-Maesano. The decrease in asthma-related mortality in France. Rev Mal Respir. 2010;27:e1-5
. DOI: 10.1016/j.rmr.2009.12.004. [PMID:20863959]
[58]
LY Wang, Y Zhong, and L Wheeler. Direct and indirect costs of asthma in school-age children. Prev Chronic Dis. 2005;2:A11 [PMID:15670464]
[59]
C Severien and HJ Friedrich. Entwicklung der Hospitalisationsraten für das Asthma bronchiale im
Kindesalter. Monatsschr Kinderheilkd. 1998;146:951-6. . Germany.DOI: 10.1007/s001120050349
[60]
J Jang, KC Gary Chan, H Huang, and SD Sullivan. Trends in cost and outcomes among adult and pediatric patients with asthma:
2000-2009. Ann Allergy Asthma Immunol. 2013;111:516
-22. DOI: 10.1016/j.anai.2013.09.007. [PMID:24267362]
[61]
FP Antunes, MDN Costa, JS Paim, LM Vieira-da-Silva, C Santos, and AA Cruz. Trends in hospitalizations for respiratory diseases in Salvador, Bahia State, Brazil,
1998-2009. Cad Saude Publica. 2012;28:869-
77. DOI: 10.1590/S0102-311X2012000500006. [PMID:22641510]
[62]
JM Chatkin, SM Barreto, NA Fonseca, CA Gutierrez, and MR Sears. Trends in asthma mortality in young people in southern Brazil. Ann Allergy Asthma Immunol. 1999;82:287
-92. DOI: 10.1016/S1081-1206(10)62610-5. [PMID:10094220]
[63]
S Cohen, N Berkman, A Avital, C Springer, L Kordoba, and Z Haklai. Decline in asthma prevalence and severity in Israel over a 10-year period.
Respiration. 2015;89:27-32
. DOI: 10.1159/000368613. [PMID:25471899]
[64]
RD Morris and RL Munasinghe. Geographic variability in hospital admission rates for respiratory disease among the
elderly in the United States. Chest. 1994;106:1172-81. DOI: 10.1378/chest.106.4.1172. [PMID:7924492]
[65]
Mathers CD, Bernard C, Iburg KM, Inoue M, Ma Fat D, Shibuya K,
et al. Global burden of disease in 2002: data sources, methods and results. Geneva: World Health
Organization; 2003.
[66]
Mathers CD, Stein C, Ma Fat D, Rao C, Inoue M, Tomijima N, et
al. Global Burden of Disease 2000: Version 2 methods and results. Geneva: World Health Organization;
2002.
[67]
B Ding, M DiBonaventura, N Karlsson, and X Ling. A cross-sectional assessment of the prevalence and burden of mild asthma in urban
China using the 2010, 2012, and 2013 China National Health and Wellness Surveys. J Asthma. 2017;54:632-43
. DOI: 10.1080/02770903.2016.1255750. [PMID:28001469]
[68]
NC Schleicher, JA Koziol, and SC Christiansen. Asthma mortality rates among California youths. J Asthma. 2000;37:259-65
. DOI: 10.3109/02770900009055448. [PMID:10831150]
[69]
J Hassanzadeh, A Mohammadbeigi, A Mousavizadeh, and M Akbari. Asthma prevalence in Iranian guidance school children, a descriptive meta-analysis.
J Res Med Sci. 2012;17:293-7
. [PMID:23267383]
[70]
PW Sullivan, VH Ghushchyan, JF Slejko, V Belozeroff, DR Globe, and SL Lin. The burden of adult asthma in the United States: Evidence from the medical
expenditure panel survey. J Allergy Clin Immunol. 2011;127:363.e1
. DOI: 10.1016/j.jaci.2010.10.042. [PMID:21281868]
[71]
AL James, MW Knuiman, ML Divitini, J Hui, M Hunter, and LJ Palmer. Changes in the prevalence of asthma in adults since 1966: the Busselton health study.
Eur Respir J. 2010;35:273-8
. DOI: 10.1183/09031936.00194308. [PMID:19643935]
[72]
D Lincoln, G Morgan, V Sheppeard, B Jalaludin, S Corbett, and J Beard. Childhood asthma and return to school in Sydney, Australia. Public Health. 2006;120:854-62
. DOI: 10.1016/j.puhe.2006.05.015. [PMID:16904142]
[74]
MA Rezvanfar, A Kebriaeezadeh, M Moein, S Nikfar, Z Gharibnaseri, and A Abdollahi-Asl. Cost analysis of childhood asthma in Iran: A cost evaluation based on referral center
data for asthma & allergies. J Res Pharm Pract. 2013;2:162-8
. DOI: 10.4103/2279-042X.128149. [PMID:24991626]
[75]
C Chouaid, A Vergnenegre, V Vandewalle, F Liebaert, A Khelifa, and Galipon. The costs of asthma in France: an economic analysis by a Markov
model. Rev Mal Respir. 2004;21:493-9
. DOI: 10.1016/S0761-8425(04)71353-4. [PMID:15292841]
[76]
SB Barnett and TA Nurmagambetov. Costs of asthma in the United States: 2002-2007. J Allergy Clin Immunol. 2011;127:145-52. DOI: 10.1016/j.jaci.2010.10.020. [PMID:21211649]
[77]
JG Trogdon, LB Murphy, OA Khavjou, R Li, CM Maylahn, and FK Tangka. Costs of Chronic Diseases at the State Level: The Chronic Disease Cost Calculator.
Prev Chronic Dis. 2015;12:E140
. DOI: 10.5888/pcd12.150131. [PMID:26334712]
[78]
M Sadatsafavi, L Lynd, C Marra, B Carleton, WC Tan, and S Sullivan. Direct health care costs associated with asthma in British Columbia. Can Respir J. 2010;17:74-80
. DOI: 10.1155/2010/361071. [PMID:20422063]
[79]
S Zannetos, T Zachariadou, A Zachariades, A Georgiou, and MA Talias. The economic burden of adult asthma in Cyprus; a prevalence-based cost of illness
study. BMC Public Health. 2017;17:262 DOI: 10.1186/s12889-017-4184-0. [PMID:28302094]
[80]
M Mukherjee, A Stoddart, RP Gupta, BI Nwaru, A Farr, and M Heaven. The epidemiology, healthcare and societal burden and costs of asthma in the UK and
its member nations: analyses of standalone and linked national databases. BMC Med. 2016;14:113 DOI: 10.1186/s12916-016-0657-8. [PMID:27568881]
[81]
D Adeloye, KY Chan, I Rudan, and H Campbell. An estimate of asthma prevalence in Africa: a systematic analysis. Croat Med J. 2013;54:519-31
. DOI: 10.3325/cmj.2013.54.519. [PMID:24382846]
[82]
CA Mendez-Luck, H Yu, YY Meng, M Jhawar, and SP Wallace. Estimating health conditions for small areas: asthma symptom prevalence for state
legislative districts. Health Serv Res. 2007;42:2389-
409. DOI: 10.1111/j.1475-6773.2007.00793.x. [PMID:17995549]
[83]
. Forecasted state-specific estimates of self-reported asthma prevalence–United
States, 1998. MMWR Morb Mortal Wkly Rep. 1998;47:1022
-5. [PMID:9853939]
[84]
IN Soyiri, DD Reidpath, and C Sarran. Forecasting peak asthma admissions in London: an application of quantile regression
models. Int J Biometeorol. 2013;57:569-
78. DOI: 10.1007/s00484-012-0584-0. [PMID:22886344]
[85]
RY Suruki, JB Daugherty, N Boudiaf, and FC Albers. The frequency of asthma exacerbations and healthcare utilization in patients with
asthma from the UK and USA. BMC Pulm Med. 2017;17:74 DOI: 10.1186/s12890-017-0409-3. [PMID:28449686]
[86]
P Lozano, P Fishman, M VonKorff, and J Hecht. Health care utilization and cost among children with asthma who were enrolled in a
health maintenance organization. Pediatrics. 1997;99:757-64
. DOI: 10.1542/peds.99.6.757. [PMID:9164766]
[87]
S Chinn, D Jarvis, P Burney, C Luczynska, U Ackermann-Liebrich, and JM Anto. Increase in diagnosed asthma but not in symptoms in the European Community
Respiratory Health Survey. Thorax. 2004;59:646-51. DOI: 10.1136/thx.2004.021642. [PMID:15282382]
[88]
H Backman, P Raisanen, L Hedman, C Stridsman, M Andersson, and A Lindberg. Increased prevalence of allergic asthma from 1996 to 2006 and further to 2016-results
from three population surveys. Clin Exp Allergy. 2017;47:1426-
35. DOI: 10.1111/cea.12963. [PMID:28608416]
[89]
S Kamble and M Bharmal. Incremental direct expenditure of treating asthma in the United States.
J Asthma. 2009;46:73-80. DOI: 10.1080/02770900802503107. [PMID:19191142]
[90]
M Wijesinghe, M Weatherall, K Perrin, J Crane, and R Beasley. International Trends in Asthma Mortality Rates in the 5-to 34-Year Age Group A Call
for Closer Surveillance. Chest. 2009;135:1045-9. DOI: 10.1378/chest.08-2082. [PMID:19349400]
[91]
T To, S Stanojevic, R Feldman, R Moineddin, EG Atenafu, and J Guan. Is asthma a vanishing disease? A study to forecast the burden of asthma in 2022.
BMC Public Health. 2013;13:254 DOI: 10.1186/1471-2458-13-254. [PMID:23517452]
[92]
J Brogger, P Bakke, GE Eide, B Johansen, A Andersen, and A Gulsvik. Long-term changes in adult asthma prevalence. Eur Respir J. 2003;21:468-72
. DOI: 10.1183/09031936.03.00056103. [PMID:12662003]
[93]
I Alvarez-Alvarez, H Niu, F Guillen-Grima, and I Aguinaga-Ontoso. Meta-analysis of prevalence of wheezing and recurrent wheezing in infants.
Allergol Immunopathol (Madr). 2018;46:210
-7. DOI: 10.1016/j.aller.2016.08.011. [PMID:27865539]
[94]
WJ Ungar, PC Coyte, KR Chapman, and L MacKeigan. The patient level cost of asthma in adults in south central Ontario. Pharmacy
Medication Monitoring Program Advisory Board. Can Respir J. 1998;5:463-71
. DOI: 10.1155/1998/362797. [PMID:10070174]
[95]
H Dai, BR Lee, and J Hao. Predicting Asthma Prevalence by Linking Social Media Data and Traditional Surveys.
Ann Am Acad Pol Soc Sci. 2017;669:75-92. DOI: 10.1177/0002716216678399
[96]
S Ram, W Zhang, M Williams, and Y Pengetnze. Predicting asthma-related emergency department visits using big data. IEEE J Biomed Health Inform. 2015;19:1216
-23. DOI: 10.1109/JBHI.2015.2404829. [PMID:25706935]
[97]
RJ Rosychuk, E Youngson, and BH Rowe. Presentations to Alberta emergency departments for asthma: a time series analysis.
Acad Emerg Med. 2015;22:942-9
. DOI: 10.1111/acem.12725. [PMID:26194859]
[98]
A Mohammadbeigi, J Hassanzadeh, and A Mousavizadeh. Prevalence of asthma in elementary school age children in Iran–a systematic
review and meta analysis study. Pak J Biol Sci. 2011;14:887-93
. DOI: 10.3923/pjbs.2011.887.893. [PMID:22518932]
[99]
M Varmaghani, F Farzadfar, F Sharifi, A Rashidian, M Moin, and M Moradi-Lakeh. Prevalence of Asthma, COPD, and Chronic Bronchitis in Iran: A Systematic Review and
Meta-analysis. Iran J Allergy Asthma Immunol. 2016;15:93
-104. [PMID:27090362]
[100]
MT Solis Soto, A Patino, D Nowak, and K Radon. Prevalence of asthma, rhinitis and eczema symptoms in rural and urban school-aged
children from Oropeza Province - Bolivia: a cross-sectional study. BMC Pulm Med. 2014;14:40 DOI: 10.1186/1471-2466-14-40. [PMID:24612913]
[101]
SY So, MMT Ng, MSM Ip, and WK Lam. Rising asthma mortality in young males in Hong Kong, 1976-85. Respir Med. 1990;84:457-61
. DOI: 10.1016/S0954-6111(08)80109-6. [PMID:2274686]
[102]
P Sullivan, VG Ghushchyan, P Navaratnam, HS Friedman, A Kavati, and B Ortiz. School absence and productivity outcomes associated with childhood asthma in the USA.
J Asthma. 2018;55:161-8. DOI: 10.1080/02770903.2017.1313273. [PMID:28453370]
[103]
JY Tzeng, CK Hsiao, and CJ Chen. Spatial model selection using Bayes factor and ratio of variabilities for asthma
mortality data. Chin J Publ Health. 1998;17:158-69.
[104]
A Entezari, Y Mehrabi, M Varesvazirian, Z Pourpak, and M Moin. A systematic review of recent asthma symptom surveys in Iranian children.
Chron Respir Dis. 2009;6:109-14
. DOI: 10.1177/1479972309103884. [PMID:19411572]
[105]
PI Frank, PD Wicks, ML Hazell, MF Linehan, S Hirsch, and PC Hannaford. Temporal change in the prevalence of respiratory symptoms and obstructive airways
disease 1993-2001. Br J Gen Pract. 2005;55:596-602
. [PMID:16105367]
[106]
TP Ng and WC Tan. Temporal trends and ethnic variations in asthma mortality in Singapore, 1976-1995.
Thorax. 1999;54:990-4. DOI: 10.1136/thx.54.11.990. [PMID:10525557]
[107]
JL López-Campos, A Cayuela, S Rodriguez-Dominguez, and E Vigil. Temporal trends in asthma mortality over 30 years. J Asthma. 2008;45:611-4. DOI: 10.1080/02770900802127006. [PMID:18773336]
[108]
H Tavakoli, JM FitzGerald, W Chen, L Lynd, T Kendzerska, and S Aaron. Ten-year trends in direct costs of asthma: a population-based study. Allergy. 2017;72:291-9. DOI: 10.1111/all.12993. [PMID:27455382]
[109]
FT Chew, DY Goh, BC Ooi, and BW Lee. Time trends and seasonal variation in acute childhood asthma in tropical Singapore.
Respir Med. 1998;92:345-50
. DOI: 10.1016/S0954-6111(98)90119-6. [PMID:9616536]
[110]
GS Graudenz, DP Carneiro, and RP Vieira. Trends in asthma mortality in the 0- to 4-year and 5- to 34-year age groups in
Brazil. J Bras Pneumol. 2017;43:24-31
. DOI: 10.1590/s1806-37562015000000253. [PMID:28380185]
[111]
D Vicendese, MJ Abramson, SC Dharmage, ML Tang, KJ Allen, and B Erbas. Trends in asthma readmissions among children and adolescents over time by age, gender
and season. J Asthma. 2014;51:1055-60
. DOI: 10.3109/02770903.2014.936447. [PMID:24949811]
[112]
P Bedouch, CA Marra, JM FitzGerald, LD Lynd, and M Sadatsafavi. Trends in Asthma-Related Direct Medical Costs from 2002 to 2007 in British Columbia,
Canada: A Population Based-Cohort Study. PLoS One. 2012;7:e50949. DOI: 10.1371/journal.pone.0050949. [PMID:23227222]
[113]
KL Chua, S Ma, S Prescott, MHK Ho, DK Ng, and BW Lee. Trends in childhood asthma hospitalisation in three Asia Pacific countries.
J Paediatr Child Health. 2011;47:723-7. DOI: 10.1111/j.1440-1754.2011.02040.x. [PMID:21999445]
[114]
S Ebmeier, D Thayabaran, I Braithwaite, C Benamara, M Weatherall, and R Beasley. Trends in international asthma mortality: Analysis of data from the WHO Mortality
Database from 46 countries (1993-2012). Lancet. 2017;390:935-45. DOI: 10.1016/S0140-6736(17)31448-4. [PMID:28797514]
[115]
J Manfreda, AB Becker, PZ Wang, LL Roos, and NR Anthonisen. Trends in physician-diagnosed asthma prevalence in Manitoba between 1980 and 1990.
Chest. 1993;103:151-7. DOI: 10.1378/chest.103.1.151. [PMID:8417870]
[116]
FJ Gonzalez-Barcala, J Aboal, JM Carreira, MX Rodriguez-Alvarez, A Puga, and E Sanjose. Trends of asthma mortality in Galicia from 1993 to 2007. J Asthma. 2012;49:1016-20
. DOI: 10.3109/02770903.2012.728272. [PMID:23102239]
[117]
C Huang, W Liu, Y Hu, Z Zou, Z Zhao, and L Shen. Updated prevalences of asthma, allergy, and airway symptoms, and a systematic review
of trends over time for childhood asthma in shanghai, China. PLoS One. 2015;10:e0121577
. DOI: 10.1371/journal.pone.0121577. [PMID:25875829]
[118]
R Newson, D Strachan, E Archibald, J Emberlin, P Hardaker, and C Collier. Acute asthma epidemics, weather and pollen in England, 1987-1994. Eur Respir J. 1998;11:694-701
. [PMID:9596123]
[119]
R Arathimos, R Granell, J Henderson, CL Relton, and K Tilling. Sex discordance in asthma and wheeze prevalence in two longitudinal cohorts.
PLoS One. 2017;12:e0176293
. DOI: 10.1371/journal.pone.0176293. [PMID:28441402]
[120]
M Hoogendoorn, TL Feenstra, and MPMH Rutten-Van Molken. Projections of future resource use and the costs of asthma and COPD in the
Netherlands. Ned Tijdschr Geneeskd. 2006;150:1243-50. . Dutch.[PMID:16796176]
[121]
AWM Suijkerbuijk, GA De Wit, AH Wijga, MJWM Heijmans, M Hoogendoorn, and MPMH Rutten-Van Molken. Societal costs of asthma, COPD and respiratory allergy. Ned Tijdschr Geneeskd. 2013;157:A6562 [PMID:24220178]
[123]
MP Rutten-van Mölken, MJ Postma, MA Joore, ML Van Genugten, R Leidl, and JC Jager. Current and future medical costs of asthma and chronic obstructive pulmonary disease
in The Netherlands. Respir Med. 1999;93:779-87
. DOI: 10.1016/S0954-6111(99)90262-7. [PMID:10603626]
[125]
D Jarvis, R Newson, C Janson, A Corsico, J Heinrich, and JM Anto. Prevalence of asthma-like symptoms with ageing. Thorax. 2018;73:37-48. DOI: 10.1136/thoraxjnl-2016-209596. [PMID:28974648]
[126]
T Nurmagambetov, R Kuwahara, and P Garbe. The Economic Burden of Asthma in the United States, 2008-2013. Ann Am Thorac Soc. 2018;15:348-
56. DOI: 10.1513/AnnalsATS.201703-259OC. [PMID:29323930]
[127]
I Aguinaga Ontoso, A Arnedo Pena, J Bellido, F Guillen Grima, and MM Suarez Varela. The prevalence of asthma-related symptoms in 13-14-year-old children from 9 Spanish
populations. The Spanish Group of the ISAAC Study (International Study of Asthma and Allergies
in Childhood)[In Spanish] Med Clin (Barc). 1999;112:171-5
. [PMID:10091210]
[128]
Y Ito, A Tamakoshi, K Wakai, K Takagi, K Yamaki, and Y Ohno. Trends in asthma mortality in Japan. J Asthma. 2002;39:633-9. DOI: 10.1081/JAS-120014928. [PMID:12442953]
[129]
A Sliwczynski, M Brzozowska, P Iltchew, T Czeleko, A Kucharczyk, and T Jedrzejczyk. Epidemiology of asthma in Poland in urban and rural areas, based on provided health
care services. Pneumonol Alergol Pol. 2015;83:178-87. [PMID:26050977]
[130]
G Luo, FL Nkoy, BL Stone, D Schmick, and MD Johnson. A systematic review of predictive models for asthma development in children.
BMC Med Inform Decis Mak. 2015;15:99 DOI: 10.1186/s12911-015-0224-9. [PMID:26615519]
[131]
HA Smit, M Pinart, JM Anto, T Keil, J Bousquet, and KH Carlsen. Childhood asthma prediction models: a systematic review. Lancet Respir Med. 2015;3:973-
84. DOI: 10.1016/S2213-2600(15)00428-2. [PMID:26597131]
[132]
SS Van Wyk, HH Lin, and MM Claassens. A systematic review of prediction models for prevalent pulmonary tuberculosis in
adults. Int J Tuberc Lung Dis. 2017;21:405-11. DOI: 10.5588/ijtld.16.0059. [PMID:28284255]
[133]
. Kwee A, Naaktgeboren CA, Franx A, Moons KGM, Koster MPH. Prediction models for the
risk of gestational diabetes: a systematic review. Diagn Progn Res. 2017;1:3 DOI:
10.1186/s41512-016-0005-7
[134]
JA Damen, L Hooft, E Schuit, TP Debray, GS Collins, and I Tzoulaki. Prediction models for cardiovascular disease risk in the general population:
systematic review. BMJ. 2016;353:i2416 DOI: 10.1136/bmj.i2416. [PMID:27184143]
[135]
ME Walsh, NF Horgan, CD Walsh, and R Galvin. Systematic review of risk prediction models for falls after stroke. J Epidemiol Community Health. 2016;70:513
-9. DOI: 10.1136/jech-2015-206475. [PMID:26767405]
[136]
G Luo, FL Nkoy, PH Gesteland, TS Glasgow, and BL Stone. A systematic review of predictive modeling for bronchiolitis. Int J Med Inform. 2014;83:691-
714. DOI: 10.1016/j.ijmedinf.2014.07.005. [PMID:25106933]
[137]
GS Collins, S Mallett, O Omar, and LM Yu. Developing risk prediction models for type 2 diabetes: a systematic review of
methodology and reporting. BMC Med. 2011;9:103 DOI: 10.1186/1741-7015-9-103. [PMID:21902820]
[138]
P Perel, P Edwards, R Wentz, and I Roberts. Systematic review of prognostic models in traumatic brain injury. BMC Med Inform Decis Mak. 2006;6:38 DOI: 10.1186/1472-6947-6-38. [PMID:17105661]
[139]
HG van den Boorn, EG Engelhardt, J van Kleef, MAG Sprangers, MGH van Oijen, and A Abu-Hanna. Prediction models for patients with esophageal or gastric cancer: A systematic review
and meta-analysis. PLoS One. 2018;13:e0192310
. DOI: 10.1371/journal.pone.0192310. [PMID:29420636]
[140]
H Squires, J Chilcott, R Akehurst, J Burr, and MP Kelly. A systematic literature review of the key challenges for developing the structure of
public health economic models. Int J Public Health. 2016;61:289-98. DOI: 10.1007/s00038-015-0775-7. [PMID:26747470]
[141]
E McManus, T Sach, and N Levell. The Use of Decision-Analytic Models in Atopic Eczema: A Systematic Review and
Critical Appraisal. Pharmacoeconomics. 2018;36:51-
66. DOI: 10.1007/s40273-017-0564-7. [PMID:28864846]
[142]
A Alsumali, A Al-Hawag, M Samnaliev, and T Eguale. Systematic assessment of decision analytic models for the cost-effectiveness of
bariatric surgery for morbid obesity. Surg Obes Relat Dis. 2018;14:1041-59. DOI: 10.1016/j.soard.2018.03.005. [PMID:29735347]
[143]
A Goehler, BP Geisler, JM Manne, B Jahn, A Conrads-Frank, and P Schnell-Inderst. Decision-analytic models to simulate health outcomes and costs in heart failure: a
systematic review. Pharmacoeconomics. 2011;29:753-
69. DOI: 10.2165/11585990-000000000-00000. [PMID:21557632]
[144]
S McLean, V Barbour, S Wild, C Simpson, and A Sheikh. Models for estimating projections for disease prevalence and burden: a systematic
review focusing on chronic obstructive pulmonary disease. J Health Serv Res Policy. 2015;20:246-53. DOI: 10.1177/1355819615579232. [PMID:25836652]
[145]
Flaxman AD, Vos DT, Murray CJ, editors. An integrative
metaregression framework for descriptive epidemiology. Seattle: University of Washington Press;
2015.
|
|
 |