Malaria is a preventable, curable but life-threatening disease caused by parasites including Plasmodium falciparum (Pf), Plasmodium vivax (Pv), Plasmodium knowlesi (Pk), Plasmodium malariae (Pm), and Plasmodium ovale (Po), of which P. falciparum and P. vivax are the most prevailing and P. falciparum the most deadly [1,2]. In 2017, there were around 219 million cases of malaria worldwide, and the attributable mortality was nearly 435 thousand [3]. Especially, children aged under 5-year-old accounted for 61% of all malaria deaths around the world, thus they are the most susceptible group affected by malaria [3,4]. Contrast to adults, children are more vulnerable to infectious diseases, and quicker differential diagnosis between malaria and non-malaria fever is needed for lessening death and severe cases.
According to World Health Organization (WHO), all suspected malaria cases should take a parasitological test to confirm the diagnosis using either microscopy or malaria rapid diagnostic tests (RDTs) [5]. The aim of this strategy is to reduce the unnecessary use of Artemisinin-based combination therapies (ACT) and prevent potential drug resistance [6]. In addition, it can improve the diagnosis of other non-malaria febrile diseases. Microscopic examination of blood slides is considered as the “gold standard” for malaria diagnosis, but it is time-consuming and requires well-trained personnel and adequate laboratory equipment, which is hard to maintain in most of the endemic areas [7,8]. RDTs can detect specific antigens produced by Plasmodium in individual blood, including histidine-rich protein-2 (HRP2), lactate dehydrogenase (LDH), and aldolase. HRP2 is specific for P. falciparum, while aldolase can be found in all species (pan-specific). LDH can be divided into three categories: Pf-specific, Pv-specific and pan-specific. Antibodies against these antigens can be combined in one type of RDTs to detect different Plasmodium species [9,10]. According to Bell and his colleagues, RDTs can be divided into 7 types depending on their target antigens (Appendix S1 in the Online Supplementary Document) [11]. Besides, pan-specific LDH only and Pv-specific LDH only tests are also available now [12]. The typical operation of RDTs is to combine a drop of finger-pricked blood and a couple of drops of buffer into RDTs cassette and wait for several minutes until the results appear on the strip. Compared to microscopy, the tests are simple to perform and interpret while providing rapid results. So, it can be used at the community level. Polymerase chain reaction (PCR) is one of nucleic acid amplification techniques, which is more sensitive than microscopy, and it can also be regarded as the “gold standard”. However, it has a higher requirement on trained technicians and standard laboratory. Thus, it does not fit the field malaria diagnosis currently and is mainly operated in epidemiological research [9,13].
Although WHO has established the diagnostic criteria, the use of parasitological tests to diagnose malaria for children was still depressed. WHO African Region accounted for 92% of all malaria cases in 2017, but according to 58 household surveys conducted in 30 sub-Saharan African countries, in 2015-2017, the median percentage of febrile children who received a diagnostic test in public health facilities was only 59% [3], which meant that there were still around two-fifths children who did not have the access to the parasitological diagnosis. Since the majority of African health facilities lack the capacity and/or device to perform microscopy [14], RDTs, which are easy to use, will be helpful to provide rapid diagnosis and avert avoidable death for children, to reach SDG 3.2 – end preventable deaths of newborns and children under-5 by 2030, and the target set by the Global Technical Strategy for Malaria 2016-2030, ie, at least 90% malaria incidence and mortality should be reduced by 2030.
The validity of RDTs has been approved in recently published systematic reviews [9,12,15], but all of them do not have restrictions on the age of the target population. The validity of RDTs in childhood malaria diagnosis has its own characteristics and may be different from adults. That is because the immunity towards Plasmodium increases with age [16], and the anti-parasite ability of children is lower than adults. It would lead to a higher parasite density of childhood malaria infection if other conditions are the same [17].
Clinical evidence has been accumulated on the operations of RDTs for childhood malaria diagnosis. However, wide disparities in their performance have been observed across studies [18-22]. These discrepancies may be attributed to different study designs, sample size, study location and reference standard used. Therefore, a systematic review was conducted to provide a comprehensive evaluation of the diagnostic accuracy and investigate the performance of RDTs against the gold standard in malaria diagnosis among children.
Search strategy and selection criteria
A systematic approach was used to search the following databases: Pubmed, Web of Science, EMBASE, Cochrane Library, the China National Knowledge Infrastructure (CNKI), Wanfang Data, and Sinomed. The latter three are Chinese databases. The search strategies were outlined in Appendix S2 in the Online Supplementary Document and no restriction was imposed. The search was undertaken on August 23, 2019, and the references of all eligible studies were checked manually to identify extra relevant articles.
The inclusion criteria were as follows: (1) Primary studies that evaluated the diagnostic accuracy of RDTs. (2) The microscopic examination of blood smears or PCR was selected as the gold standard. (3) Participants were children. (4) Studies that reported the direct comparison results between RDTs and the gold standard. The exclusion criteria were as follows: (1) Studies that were case reports, reviews, editorials, letters, comments, and conference abstracts. (2) Studies that did not present enough information to extract or calculate the number of true-positives, false-positives, true-negatives, and false-negatives. The title and abstract of all relevant articles were read by three reviewers independently according to the inclusion and exclusion criteria during the first round of screening. Then the full texts of the eligible studies were rechecked based on the same criteria. Any disagreement between three reviewers was resolved by discussion.
Data extraction and quality assessment
The following data were independently extracted by three reviewers from eligible studies using Microsoft Excel 2016 (Microsoft Inc, Seattle WA, USA): (1) study characteristics: journal, publication year, first author and his/her institution, study period, study setting, and study design. (2) participants’ characteristics: the inclusion and exclusion criteria, sample size, the number of malaria cases, the age range and sex distribution of participants, and the parasite density of Plasmodium. (3) RDTs characteristics: commercial brand, and specific Plasmodium species and antigens detected. (4) RDTs performance: the reference standard, and the number of true-positives, false-positives, true-negatives, and false-negatives. Any discrepancy between three reviewers was resolved by discussion. If only a subset of participants met the selection criteria, data were extracted only for the subgroup.
The revised Quality Assessment of Diagnostic Accuracy Studies (QUADAS-2) tool was used to assess the methodological quality of the eligible studies [23], as recommended by the Cochrane Collaboration. The tool has four domains: patient selection, index test, reference standard, and flow and timing. Each domain was scored as “high/low risk of bias” and “high/low applicability concern”, except for the last one which only contains the risk of bias section. If insufficient data were reported, the corresponding section would be classified as “unclear”. Reviewers assessed the quality of studies independently using Review Manager 5.3 and discussed the inconsistencies. The criteria for each section are listed in Appendix S3 in the Online Supplementary Document.
Statistical analysis
We estimated the sensitivity and specificity of each study with 95% confidence intervals (CI) and presented the results in forest plots. Then we used the Midas module in Stata 12.1 (StataCorp LLC, Texas, USA) to calculate the pooled estimates of the sensitivity, specificity, positive likelihood ratio, negative likelihood ratio, and diagnostic odds ratio. Midas is a comprehensive program for undertaking meta-analysis of diagnostic test accuracy in Stata. Its primary data synthesis is based on the bivariate mixed-effects regression framework. A hierarchical summary receiver operating characteristic curve (HSROC) was fitted and funnel plots were presented respectively to show the comprehensive diagnostic value of RDTs and the potential publication bias among eligible studies. Compared with the summary receiver operating characteristic (SROC) model, the HSROC model allows more between and within-study variability [24]. It was adopted as studies included are expected to show considerable heterogeneity in diagnostic accuracy [25]. We also performed the Q test to assess the heterogeneity among the included studies. The extent of heterogeneity was quantified by I2 measure [26]. If the heterogeneity was significant (I2>50%), we used Meta-disc 1.4.0 software (the Unit of Clinical Biostatistics team of the Ramón y Cajal Hospital, Madrid, Spain) to explore whether a threshold effect existed. Furthermore, meta-regression was conducted to investigate the potential sources of heterogeneity. Covariates included local malaria transmission type, study design, sampling method, reference standard, sample size, geographic location, blinding status, RDTs type, and target antigens. In the regression, the accuracy measure was relative diagnostic odds ratio (RDOR). The coefficients of covariates indicated the change in the diagnostic performance of the RDTs under each study per unit increase in the covariates. In other words, P < 0.05 represented that the corresponding covariates were the major sources of heterogeneity. Subgroup analyses based on the sources of heterogeneity were subsequently conducted by Stata (Stata Corp, College Station, TX, USA).
For meta-regression and subgroup analyses, transmission type was divided into four categories: high, low-to-moderate, mixed and unclear. The transmission type was classified as “high” if the authors described it as “hyperendemic”, “perennial”, “holoendemic” or “high”; “low-to-moderate” when it was “mesoendemic”, “sporadic”, “moderate” or “low”; “mixed” if it was described as “seasonal” or when multiple sites of different transmission types were included; “unclear” if the transmission type was not mentioned.
Results of the search
A total of 9731 relevant articles were identified. After removing duplicates, 5933 articles were selected. 5861 articles were excluded based on the criteria. The full texts of 72 articles were evaluated and 51 of them were eventually included. Among them, the most common reason for exclusion was the lack of data for a 2x2 table. The detailed process for selection is shown in Figure 1.
Out of 51 studies included in the review, two evaluated the validity of RDTs when they were used to monitor the effects of ACT treatment, and the others assessed the diagnostic capacity of RDTs. Since HRP2 will be cleared slowly from bloodstream if the treatment of P. falciparum is successful and it can contribute to a higher false-positive rate of RDTs [27], our analyses were grouped into two parts according to the usage time of RDTs (before or after ACT treatment). There were 9 studies that adopted multiple types of RDTs and/or reference standards: 6 studies evaluated 2 types of RDTs [28-33], 2 studies evaluated 3 types of RDTs [18,34], and 2 studies selected two different types of reference standards [32,35]. Particularly, in one study, two staffs read the RDTs strips respectively, so there were two different results for each type of RDTs [29]. As enrolled children were asked to retest blood samples during the follow-up regularly, the studies that assessed the RDTs capacity for monitoring the effect of ACT treatment all have multiple test evaluations. As a result, we had 82 test evaluations reporting a total of 57 312 test results. Among them, 34.45% of tests (19 746) showed the positive result against the reference standard. Most of the studies included were conducted in Africa (n = 47), and the rest of them happened in Asia (three in India and one in Pakistan). The detailed characteristics of the included studies were summarized in Table 1.
Study | Country* | Transmission type* | Study design | Sample size | Children age range* | Sex (M/F)* | Parasite density range (parasites/μl)* | RDTs target antigens* | Reference standard* | |
---|---|---|---|---|---|---|---|---|---|---|
1 | Singh, 2002 [36] | India | Mesoendemic | Cross-sectional study | 573 | 5Y-14Y | / | 78-6360 | HRP2 | Microscopy |
2 | Mtove, 2011 [19] | Tanzania | Perennial | Prospective cohort | 965 | 3M-59M | / | / | HRP2 | Microscopy |
3 | Shaikh, 2013 [37] | Pakistan | Moderate | Cross-sectional study | 400 | 2M-5Y | 140/260 | / | / | Microscopy |
4 | Keating, 2009 [20] | Zambia | / | Cross-sectional study | 618 | 0M-59M | 324/294 | 6-678 | HRP2 | Microscopy |
5 | Owusu, 2018 [38] | Ghana | Perennial | Cross-sectional study | 401 | ≤5Y | 98/303 | / | HRP2 | PCR |
6 | Kashosi, 2017 [39] | DR Congo | Sporadic & seasonal | Cross-sectional study | 460 | 6M-59M | 235/225 | / | HRP2 & pan-specific LDH | Microscopy |
7 | Ayeh, 2011 [40] | Ghana | / | Cross-sectional study | 200 | 4D-5Y | / | / | Pf-specific LDH & pan-specific LDH | Microscopy |
8 | Kiemde, 2017 [41] | Burkina Faso | / | Cross-sectional study | 684 | <5Y | 369/314 | / | HRP2 | Microscopy |
9 | Baiden, 2012 [42] | Ghana | / | Prospective cohort | 436 | 3M-60M | 236/200 | 31-1 518 575 | HRP2 | Microscopy |
10 | Gerstl, 2010 [28] | Sierra Leone | Hyperendemic | Prospective cohort | 343 | 2M-58M | 177/166 | 1-2 136 000 | Pf-specific LDH & pan-specific LDH; HRP2 | Microscopy |
11 | Premji, 1994 [43] | Tanzania | / | Cross-sectional study | 380 | ≤42M | / | / | HRP2 | Microscopy |
12 | Singh, 2001 [44] | India | / | Cross-sectional study | 191 | 2Y-10Y | / | / | HRP2 | Microscopy |
13 | Nkrumah, 2011 [6] | Ghana | / | Cross-sectional study | 263 | 3Y-16Y | 147/116 | 50-8320 | HRP2 & aldolase | Microscopy |
14 | Hopkins, 2007 [29] | Uganda | / | Prospective cohort | 918 | 1.5Y-11.5Y | / | / | HRP2; only pan-specific LDH | Microscopy |
15 | Adesanmi, 2011 [45] | Nigeria | / | Cross-sectional study | 380 | 6M-59M | / | / | HRP2 | Microscopy |
16 | Bojang, 1999 [46] | Gambia | High | Cross-sectional study | 139 | / | / | / | HRP2 | Microscopy |
17 | Hendriksen, 2011 [30] | Tanzania; Mozambique | High; Lower | Cross-sectional study | 1898 | / | 990/908 | / | HRP2; Pf-specific LDH & pan-specific LDH | Microscopy |
18 | Rabiu, 2013 [21] | Nigeria | / | Cross-sectional study | 209 | 6M-12Y | 106/103 | 40-203 883 | HRP2 | Microscopy |
19 | Mens, 2007 [34] | Kenya; Tanzania | High; Mesoendemic | Cross-sectional study | 338 | 6M-12Y | 191/147 | / | Pf-specific LDH & pan-specific LDH; HRP2; HRP2 & pan-specific LDH | Microscopy |
20 | Kamugisha, 2008 [47] | Tanzania | / | Cross-sectional study | 301 | / | 163/138 | / | HRP2 | Microscopy |
21 | Valéa, 2009 [48] | Burkina Faso | Hyperendemic | Cross-sectional study | 464 | 6M–59M | / | 40-234 280 | Pf-specific LDH & pan-specific LDH | Microscopy |
22 | Hawkes, 2014 [49] | Uganda | / | Cross-sectional study | 2000 | 2M-5Y | 1114/886 | / | HRP2 & pan-specific LDH | Microscopy |
23 | Oyeyemi, 2016 [22] | Nigeria | / | Cross-sectional study | 200 | / | 102/98 | / | HRP2 | Microscopy |
24 | Mahende, 2016 [50] | Tanzania | Low to moderate | Cross-sectional study | 867 | 2M-59M | 459/408 | / | HRP2 | PCR |
25 | Ilombe, 2014 [51] | DR Congo | / | Cross-sectional study | 872 | / | 469/403 | / | HRP2 & pan-specific LDH | Microscopy |
26 | Ajumobi, 2015 [52] | Nigeria | Mesoendemic; Seasonal | Cross-sectional study | 300 | 6–59M | 163/132 | / | HRP2 | Microscopy |
27 | Sotimehin, 2007 [53] | Nigeria | / | Cross-sectional study | 205 | 0D–3D | / | 17-2940 | Pf-specific LDH & pan-specific LDH | Microscopy |
28 | Tarimo, 2015 [54] | Tanzania | Intensive perennial | Cross-sectional study | 474 | 0-59M | 246/220 | / | HRP2 & pan-specific LDH | Microscopy |
29 | Ratsimbasoa, 2012 [35] | Madagascar | High; Low | Cross-sectional study | 543 | 2-59M | - | 48-82 000 | HRP2 & pan-specific LDH | PCR; Microscopy |
30 | Wanji, 2008 [55] | Cameroon | Hyperendemic | Cross-sectional study | 186 | 4Y-16Y | 88/98 | / | only pan-specific LDH | Microscopy |
31 | Tiono, 2013 [56] | Burkina Faso | Seasonality | Cross-sectional study | 525 | 6M-59M | 248/277 | / | HRP2 | Microscopy |
32 | Swarthout, 2007 [57] | DR Congo | High; Seasonal | Cross-sectional study | 358 | 6M-59M | / | / | HRP2 | Microscopy |
33 | Samadoulougou, 2014 [58] | Burkina Faso | / | Cross-sectional study | 6260 | 6M-59M | 3181/3079 | / | HRP2 | Microscopy |
34 | Grandesso, 2016 [18] | Uganda | Low; High | Prospective cohort | 5262 | / | 2711/2551 | / | HRP2; HRP2; only pan-specific LDH | Microscopy |
35 | Venkatesh, 2007[59] | India | / | Cross-sectional study | 149 | ≤12Y | / | / | HRP2 | Microscopy |
36 | Bouyou, 2013 [31] | Gabon | Perennial | Cross-sectional study | 386 | ≤10Y | / | / | HRP2 & aldolase; HRP2 | Microscopy |
37 | Aydin, 2013 [60] | Tanzania | Moderately high | Prospective cohort | 53 | 10M-59M | 35/18 | 2000-250 000 | HRP2 | PCR |
38 | Houze, 2009 [33] | Benin | High; Seasonal | Prospective cohort | 205 | 6M-59M | / | 1000-525 000 | HRP2; Pf-specific LDH & pan-specific LDH | Microscopy |
39 | Adebisi, 2018 [61] | Nigeria | .. | Cross-sectional study | 370 | <5Y | 211/159 | / | HRP2 | Microscopy |
40 | Al, 2019 [62] | Uganda | Mixed | Cross-sectional study | 247 | .. | 124/123 | / | HRP2 & pan-specific LDH | PCR |
41 | Bloch, 2018 [63] | Tanzania | .. | Cross-sectional study | 1049 | 1M-59M | 527/503 | / | / | Microscopy |
42 | Dada, 2018 [64] | Nigeria | holoendemic | Cross-sectional study | 102 | 7M-17Y | 58/44 | / | HRP2 | Microscopy |
43 | Nkefou, 2018 [65] | Cameroon | .. | Cross-sectional study | 249 | 6M-15Y | 134/115 | / | HRP2 & pan-specific LDH | Microscopy |
44 | Hofmann, 2019 [66] | Tanzania | Low | Cross-sectional study | 3192 | 2M-59M | 1766/1426 | / | HRP2 | PCR |
45 | Iwuafor, 2018 [67] | Nigeria | High | Cross-sectional study | 270 | 1M-59M | / | / | HRP2 | Microscopy |
46 | Kiemde, 2019 [68] | Burkina Faso | Seasonal | Cross-sectional study | 407 | ≤5Y | 231/176 | / | HRP2 & pan-specific LDH | Microscopy |
47 | Kiemde, 2018 [69] | Burkina Faso | Seasonal | Cross-sectional study | 407 | ≤5Y | 231/176 | 32–58 625 | HRP2 | Microscopy |
48 | Kitutu, 2018 [70] | Uganda | Low | Cross-sectional study | 212 | 2M-60M | 106/95 | / | HRP2 | PCR |
49 | Peprah, 2019 [71] | Tanzania | Mixed | Cross-sectional study | 819 | 0Y-15Y | 432/387 | / | HRP2 | Microscopy |
50 | Quakyi, 2018 [32] | Ghana | Mixed | Prospective cohort | 260 | <5Y | 131/129 | 300-99 500 | HRP2; HRP2 & pan-specific LDH | Microscopy; PCR |
51 | Teh, 2019 [72] | Cameroon | perennial | Cross-sectional study | 491 | 6M-14Y | 218/273 | 70-1162 | HRP2 | Microscopy |
RDTs – malaria rapid diagnostic tests, Y – years, M – months, D – days, M – male, F – female, HRP2 – histidine-rich protein-2, LDH – lactate dehydrogenase, Pf – Plasmodium falciparum, Pan - all Plasmodium species, PCR – polymerase chain reaction, DR Congo – The Democratic Republic of the Congo, / – missing data, & – and
*Semicolons separate different test evaluations.
The methodological quality of the included studies
The overall methodological quality of studies included was relatively high, as the scores of risk of bias and applicability concerns in the four domains were mainly low. Most studies (n = 41) enrolled consecutive or random sample of patients. None of them were case-control studies. 17 studies adopted double-blind method, and 1 study was single-blind, but the rest did not supply sufficient information. 6 studies selected PCR as the reference standard, 43 studies took microscopy as the reference standard, and 2 studies chose both. 31.37% (16/51) of the eligible studies were scored to have a high risk of bias in the flow and timing domain since they did not include all patients in the analysis. 35.29%(18/51) of included studies had a high applicability concern in patient selection domain because of their unrepresentative samples. The results of the methodological quality were shown in Figure 2, Panel A and Panel B.
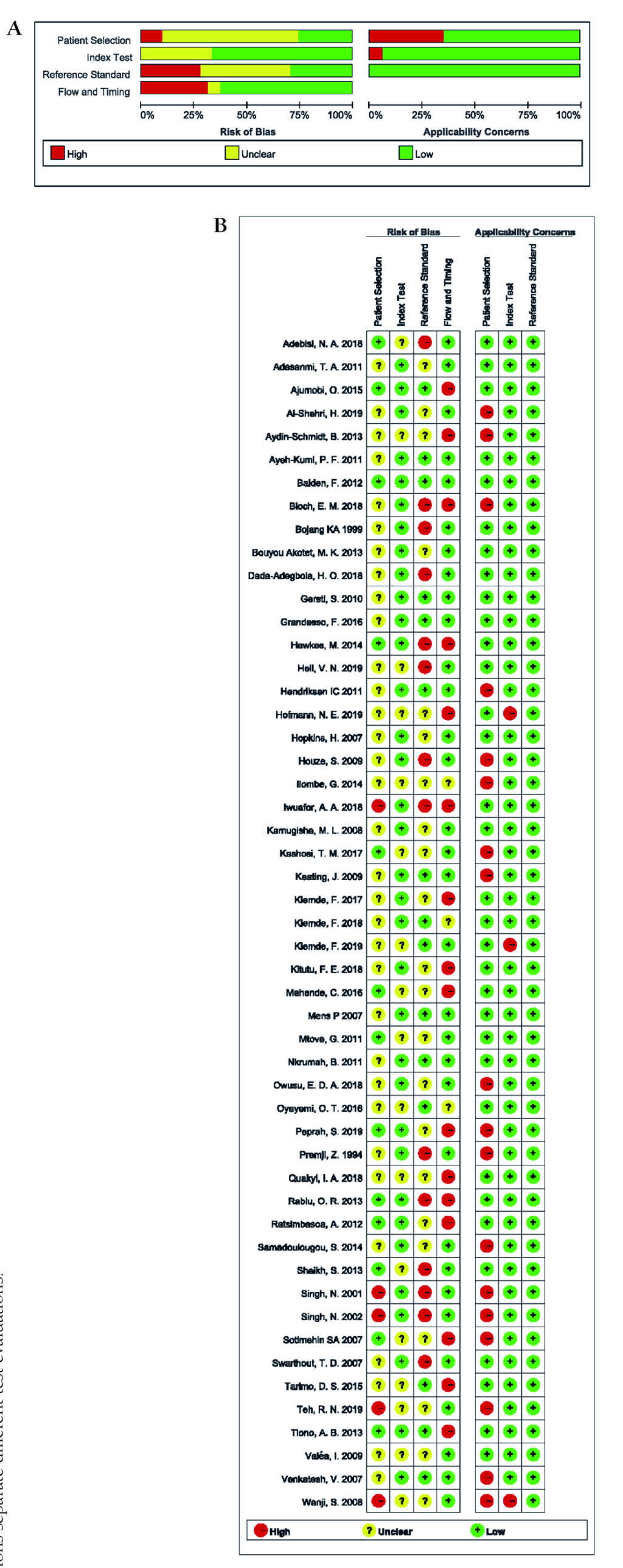
Diagnostic accuracy of RDTs
There were 63 test evaluations that focused on the diagnostic accuracy of RDTs [6,18-22,28-32,34-59,61-72]. 59 were conducted in Africa and 4 in Asia. 55 tests selected microscopy as the reference standard and 8 chose PCR. The median sample size was 400 (range: 102 - 6260). 14 tests evaluated the performance of RDTs in a high malaria transmission setting, 6 in low-to-moderate and 20 in mixed.
Sensitivities of tests ranged from 0.00 to 1.00, and specificities from 0.08 to 1.00 (Figure 3). The pooled summary of sensitivity and specificity (95% CI) of RDTs were 0.93 (0.90-0.95) and 0.93 (0.90-0.96) respectively. The pooled estimates for the positive likelihood ratio, negative likelihood ratio, and diagnostic odds ratio (95% CI) were 13.67 (8.94-20.90), 0.07 (0.05-0.10), and 192.67 (111.46-333.06) respectively. HSROC curve summarized the sensitivity and specificity of RDTs in Figure 4. The area under the curve is close to 100%, indicating that the performance of RDTs was satisfactory. High heterogeneity was observed between studies (Cochrane’s Q = 2182.22, I2 = 100.00, P < 0.001), thus we explored its source through the threshold effect analysis and meta-regression. The results suggested that there was no threshold effect between studies (P = 0.06), while transmission type, sampling method and study design were the major sources of heterogeneity (P < 0.05). The results of the meta-regression were shown in Table 2. In addition, the effect of each variable on the accuracy of RDTs was presented by forest plots if the variable was categorical (Figure 5, Panel A and Panel B), and by scatter plots if it was continuous (Figure 6, Panel A and Panel B).
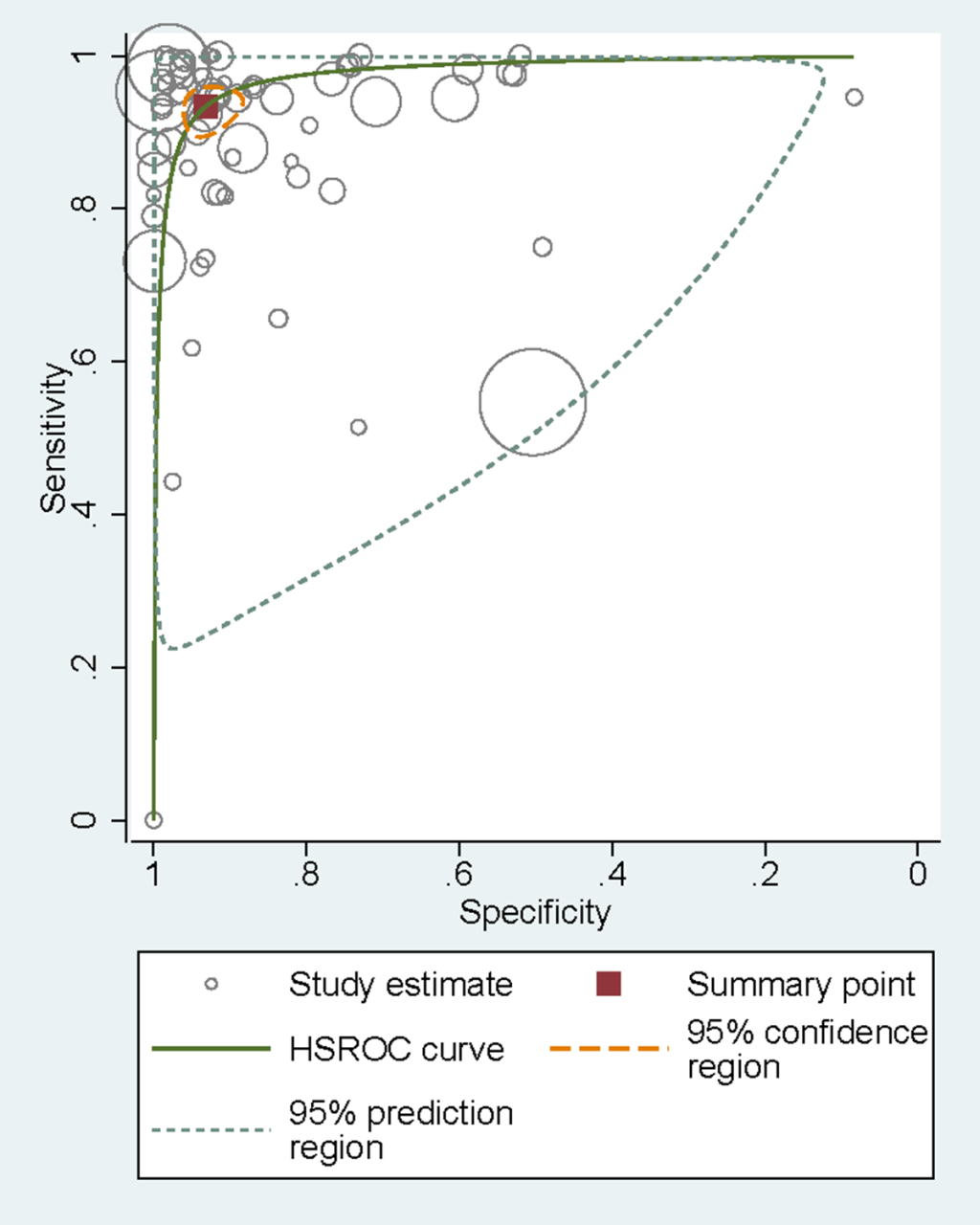
Coefficient | Standard error | P-value | RDOR | 95% CI | |
---|---|---|---|---|---|
Cte | 7.83 | 2.23 | 0.00 | NA | NA |
S | -0.40 | 0.12 | 0.00 | NA | NA |
Transmission type | -0.46 | 0.16 | 0.00 | 0.63 | 0.46-0.86 |
Study design | 1.18 | 0.58 | 0.05 | 3.24 | 1.01-10.45 |
Sampling method | -2.16 | 0.83 | 0.01 | 0.12 | 0.02-0.61 |
RDTs type | -0.23 | 0.25 | 0.38 | 0.80 | 0.48-1.32 |
Reference standard | -1.32 | 0.74 | 0.08 | 0.27 | 0.06-1.19 |
HRP2 based or not | 0.49 | 0.88 | 0.58 | 1.63 | 0.28-9.51 |
Sample size | 0.00 | 0.00 | 0.05 | 1.00 | 1.00-1.00 |
Continent | 1.57 | 1.11 | 0.16 | 4.82 | 0.52-44.32 |
Blinding status | -0.33 | 0.28 | 0.25 | 0.72 | 0.41-1.27 |
RDTs – malaria rapid diagnostic tests, HRP2 – histidine-rich protein-2, NA – not applicable, CI – confidence interval, RDOR – relative diagnostic odds ratio, Cte – constant term in the equation, S – a measure of threshold
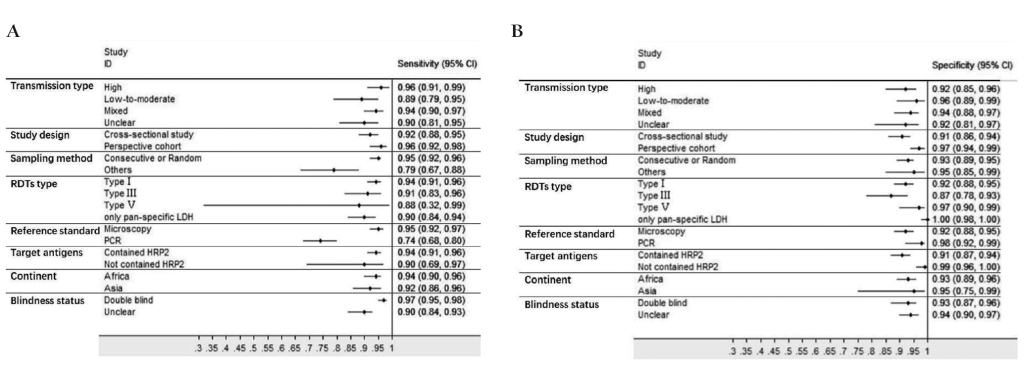
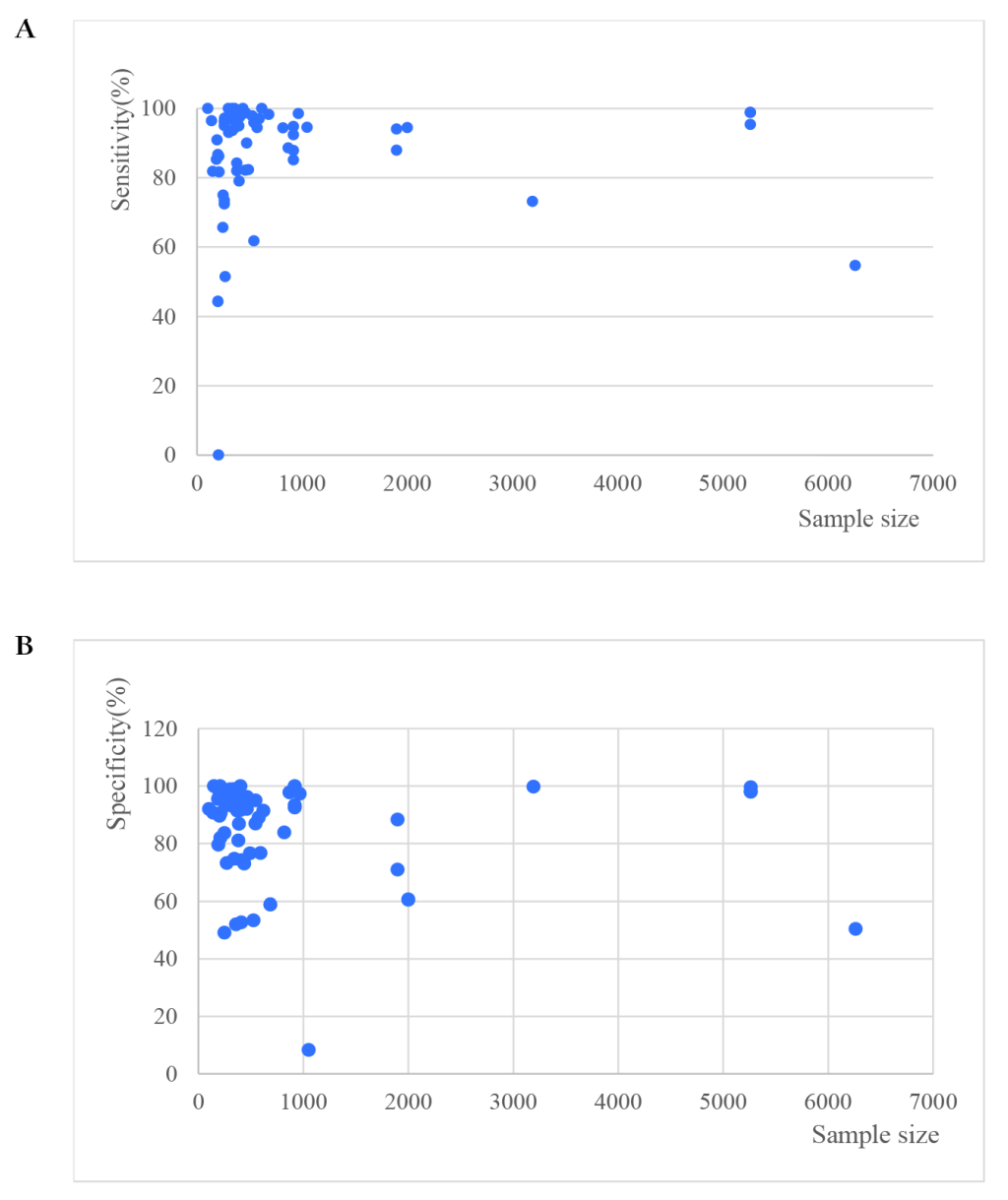
Subgroup analyses were conducted and the results were shown in Table 3. In brief, RDTs conducted in high malaria transmission areas had higher sensitivity but lower specificity compared to low-to-moderate areas. The studies with consecutive or random sample of patients presented higher sensitivity than others. Both sensitivity and specificity estimated by prospective cohort studies appeared to be higher in comparison with cross-sectional studies. A funnel plot was presented in Figure 7. It demonstrated the existence of publication bias (P = 0.04), and it was found that studies with high accuracy results tended to be published.
Category | No. of tests | Sensitivity (95% CI) | Specificity (95% CI) | Positive likelihood ratio (95% CI) | Negative likelihood ratio (95% CI) | Diagnostic odds ratio (95% CI) | |
---|---|---|---|---|---|---|---|
Transmission type | High | 14 | 0.96 (0.91-0.99) | 0.92 (0.85-0.96) | 12.7 (6.4-25.3) | 0.04 (0.02-0.10) | 323 (103-1005) |
Low-to-moderate | 6 | 0.89 (0.79-0.95) | 0.96 (0.89-0.99) | 25.3 (7.7-83.0) | 0.11 (0.06-0.22) | 223 (66-759) | |
Mixed | 20 | 0.94 (0.90-0.97) | 0.94 (0.88-0.97) | 15.0 (8.0-28.2) | 0.06 (0.03-0.10) | 254 (104-618) | |
Unclear | 23 | 0.90 (0.81-0.95) | 0.92 (0.81-0.97) | 11.5 (4.6-28.5) | 0.11 (0.06-0.20) | 107 (39-290) | |
Sampling method | Consecutive or random | 54 | 0.95 (0.92-0.96) | 0.93 (0.89-0.95) | 13.0 (8.3-20.5) | 0.06 (0.04-0.09) | 226 (125-409) |
Others | 9 | 0.79 (0.67-0.88) | 0.95 (0.85-0.99) | 16.8 (5.0-56.5) | 0.22 (0.13-0.36) | 78 (20-294) | |
Study design | Cross-sectional study | 48 | 0.92 (0.88-0.95) | 0.91 (0.86-0.94) | 10.2 (6.4-16.1) | 0.09 (0.06-0.13) | 115 (64-207) |
Prospective cohort | 15 | 0.96 (0.92-0.98) | 0.97 (0.94-0.99) | 34.4 (15.6-75.9) | 0.04 (0.02-0.08) | 897 (359-2242) | |
HRP2 based or not | Contained HRP2 | 51 | 0.94 (0.91-0.96) | 0.91 (0.87-0.94) | 10.9 (7.4-16.0) | 0.07 (0.05-0.10) | 158 (90-278) |
Not contained HRP2 | 10 | 0.90 (0.69-0.97) | 0.99 (0.96-1.00) | 85.9 (21.9-336.7) | 0.10 (0.03-0.35) | 847 (194-3704) | |
Unclear | 2 | / | / | / | / | / |
CI – confidence interval, HRP2 – histidine-rich protein-2, /– did not have enough test evaluations to perform the meta-analysis
Many studies discussed the diagnostic value of HRP2 based RDTs vs LDH based RDTs [73,74], and the problem about which type of RDTs is better still exists. Although target antigens were not the major sources of heterogeneity, a subgroup analysis was performed based on it. Results showed that HRP2 based RDTs had higher sensitivity but lower specificity than RDTs that did not contain HRP2 (Table 3). But there was no statistically significant difference.
RDTs capacity for monitoring the effect of ACT treatment
19 tests evaluated RDTs’ capacity of monitoring the effect of ACT treatment [33,60]. All were conducted in Africa. Fourteen tests took microscopy as the reference standard and five took PCR. Since the days after initial treatment is an important factor affecting the accuracy of RDTs, we analyzed the results based on this framework. Consequently, after categorizing based on the follow-up period, there was no more than 3 tests within each category, and thus we could not perform a meta-analysis (To perform Midas, a minimum of four 2 × 2 tables is required). In the lack of statistical pooling, we presented the findings in a narrative table (Table 4). In short, the specificity of HRP2 based RDTs increased with the follow-up period. And at early stages after the initial treatment, the specificity of Pf-LDH based RDTs was much higher than HRP2 based RDTs.
RDTs target antigens | Days after initial treatment | TP | TN | FP | FN | Sensitivity (%) | Specificity (%) | |
---|---|---|---|---|---|---|---|---|
Aydin-Schmidt, 2013 [60] | HRP2 | Day 14 | 3 | 8 | 32 | 0 | 100.00 | 20.00 |
Day 21 | 1 | 15 | 27 | 0 | 100.00 | 35.71 | ||
Day 28 | 1 | 24 | 18 | 0 | 100.00 | 57.14 | ||
Day 35 | 1 | 32 | 10 | 0 | 100.00 | 76.19 | ||
Day 42 | 2 | 38 | 3 | 0 | 100.00 | 92.68 | ||
Houze, 2009 [33] | HRP2 | Day 3 | 35 | 28 | 134 | 0 | 100.00 | 17.28 |
Day 7 | 6 | 49 | 115 | 1 | 85.71 | 29.88 | ||
Day 14 | 6 | 87 | 69 | 1 | 85.71 | 55.77 | ||
Day 21 | 14 | 104 | 38 | 2 | 87.50 | 73.24 | ||
Day 28 | 13 | 92 | 63 | 2 | 86.67 | 59.35 | ||
Day 35 | 9 | 79 | 4 | 2 | 81.82 | 95.18 | ||
Day 42 | 2 | 73 | 4 | 1 | 66.67 | 94.81 | ||
Houze, 2009 [33] | Pf-specific LDH & pan-specific LDH | Day 3 | 28 | 141 | 21 | 7 | 80.00 | 87.04 |
Day 7 | 5 | 151 | 13 | 2 | 71.43 | 92.07 | ||
Day 14 | 5 | 150 | 6 | 2 | 71.43 | 96.15 | ||
Day 21 | 16 | 137 | 5 | 0 | 100.00 | 96.48 | ||
Day 28 | 13 | 122 | 3 | 2 | 86.67 | 97.60 | ||
Day 35 | 9 | 81 | 2 | 2 | 81.82 | 97.59 | ||
Day 42 | 2 | 77 | 0 | 1 | 66.67 | 100.00 |
RDTs – malaria rapid diagnostic tests, HRP2 – histidine-rich protein-2, LDH – lactate dehydrogenase, Pf – Plasmodium falciparum, Pan - all Plasmodium species, TP – true-positives, TN – true-negatives, FP – false-positives, FN – false-negatives
Our results demonstrated that RDTs had relatively high sensitivity and specificity for malaria diagnosis in children and all the findings were reported based on the PRISMA Checklist (Appendix S4 in the Online Supplementary Document) [75]. Since there is no previous systematic review focused on children, the results were compared with those of the whole population. Abba’s research found that the sensitivity of RDT varied between 0.915 and 0.995, while its specificity ranged from 0.906 and 0.987[9], which is comparable to our results. Moreover, in high transmission areas, the sensitivity and specificity were higher among children (0.96 and 0.92, respectively) than the whole population (0.937 and 0.896, respectively) [9]. This may relate to the fact that because adults have greater immune status than children, adult patients with malaria is more likely to have lower parasite density [60,76], and it could be difficult for RDTs to detect the low concentration of antigens among them. This characteristic makes RDTs more suitable for childhood malaria detection in high transmission areas. Another research conducted by Li calculated the accuracy of HRP2 based RDTs [15]. Comparatively, it had lower sensitivity (0.94 vs 0.96, respectively) but higher specificity (0.91 vs 0.86, respectively) in children than in adults.
Besides high diagnostic accuracy, RDTs also have the advantages of rapid detection and are easy-to-use, making it feasible to utilize it at primary health care centers. These advantages can be particularly important for P. falciparum detection, as it can progress rapidly from an uncomplicated febrile illness to potentially deadly disease [77]. Furthermore, compared to microscopy or PCR, the diagnostic cost of RDTs is relatively low, with a low cost of RDT strips and the training fees for laboratory staff. A few studies have been undertaken to evaluate the economic value of RDTs and they demonstrated that in comparison with microscopy, RDTs are more cost-effective if the whole treatment course have been taken into account [78-80]. Therefore, as most of the malaria-endemic areas have limited resources, RDTs is of high value to be used there. For instance, in a large proportion of African lower-level health facilities, technical expertise and microscopy were not available for children [81]. Likewise, almost half of the suspected malaria patients seek care in the private sector in Africa [82], which could be even less equipped.
Considering the endemicity of malaria, RDTs performed in high transmission areas had higher sensitivity but lower specificity than those conducted in low-to-moderate areas. This may be because low-density infection represents a significant proportion of malaria infections among children in low-transmission settings [83,84], leading to a higher false-negative rate. Furthermore, for HRP2 based RDTs, the remaining HRP2 antigen will last for several weeks in peripheral blood after a successful treatment, leading to false-positive results [27,57]. This is more common in high transmission areas since the children there may be infected with P. falciparum several times across their lives [28,60].
Though we did not impose any restriction on the country or region, only four studies conducted in Asia were included, and the rest of them were all performed in Africa. However, each endemic area has its own epidemiological characteristics, and the evidence of Africa cannot verify the applicability of RDTs in other areas. For instance, in the WHO South-East Asia Region, where the incidence rate was 7.0 per 1000 population at risk in 2017, both P. falciparum and P. vivax were dominant parasites [3,85]. P. knowlesi infection was also widely distributed there [86]. Meanwhile, most countries are confronted with the problem of limited resource. For example, India carries a high proportion of disease burden, however, microscopies were not accessible for suspected children in poor, remote villages [36,44]. Another endemic area is the WHO Americas Region, where the incidence rate was 7.3 per 1000 population at risk in 2017 [3]. Evidence demonstrated that a large proportion of P. falciparum lacked pfhrp2 or pfhrp3 or both genes there [87], which may lead to invalidity of HRP2 based RDTs. Therefore, corresponding research conducted in these areas is urgently needed.
There are two limitations to be considered in this study. First, since the parasite density of patients is a critical factor for the sensitivity of RDTs, we intended to perform a subgroup analysis. However, almost half of the included studies did not report the geometric mean parasite densities of patients, so linear regression could not be performed. Furthermore, it seemed that there was no widely-recognized standard for the classification of Plasmodium parasite density, and most of the studies classified it differently. Also, because none of the studies provided individual-level data, we could not classify the parasite density by ourselves. As a result, we could not add this factor into meta-regression and subgroup analyses, which might introduce bias. Second, our findings may be more transferable to Africa as most of the included studies were conducted there.