Diarrhoea is a major cause of morbidity and mortality in lower and middle income countries with the primary treatment being rehydration regimens matched to the severity of the dehydration [1,2]. Co-treatment with zinc supplements is also recommended [2,3]. This is based on results of randomised controlled trials and subsequent systematic reviews [4-8] suggesting that zinc supplements reduce diarrhoea duration for children aged six months and above. Kenyan guidelines in keeping with those of the World Health Organisation [WHO] recommend treatment of diarrhoea with fluids and co-treatment with oral zinc for 14 days for all children irrespective of illness severity [2].
Despite these recommendations, there remains some debate on the benefits of zinc since:
-
The prevalence of zinc deficiency varies by settings [9] and this may contribute to between country variations in zinc effectiveness reported in a systematic review by Patel (2012) [10].
-
Trials supporting the use of zinc have included fewer participants from Africa than other low and middle income settings [7] and it is suggested that African children with diarrhoea have poor health outcomes [1].
-
There are few data supporting the benefits of zinc in children younger than six months and few data on the effects of zinc in children of all ages who are relatively well-nourished [7].
Conducting trials to address all these questions would likely be expensive and time-consuming. Appropriate analyses of observational data sets may help address such questions while also providing data on the effectiveness of zinc treatment in non-trial populations.
Objectives
The primary focus of this analysis is to examine the effectiveness of zinc supplementation, assuming those prescribed zinc actually received it, in reducing time to discharge for children admitted with diarrhoea in Kenyan hospitals. In secondary analyses, we aim to examine the effectiveness of zinc amongst those classified as either severely-moderately under-nourished or well-nourished.
Study design and data source
We use observational data from the Kenyan Clinical Information Network (CIN) that was initiated in September 2013 to improve inpatient paediatric data availability from 14 hospitals. These hospitals typically have one paediatrician leading services predominantly provided by junior clinical teams. Data in these hospitals are collected post discharge by trained data clerks guided by well-defined standard operating procedures, under supervision by the hospital medical records department and the research team. Clinicians admitting patients fill standardized Paediatric Admission Record (PAR) forms [11] that have been shown to improve documentation of clinical symptoms and signs [12]. Together with discharge forms, treatment sheets and laboratory reports these are all part of the patient files that are the primary data source. Information on zinc prescription was abstracted from the treatment sheets when clinicians indicated to have prescribed this treatment. The data used for this analysis are those collected between October 2013 and February 2017. More information on CIN is provided in the supplementary material.
Statistical analysis
Analysis populations
We include in the analysis all children who had diarrhoea as one of the illnesses on admission. We exclude children aged <1 month and ≥60 months, had shock or who had impaired consciousness (response only to pain or unresponsive), and those who had a clinical diagnosis of severe acute malnutrition. We primarily examine the effect on time to discharge of whether children were prescribed zinc in two age groups: all children aged 1-5 months (group 1) and all children aged 6-59 months (group 2). The analysis is stratified into two age groups as these are the age groups considered in the guidelines, with different zinc prescription recommendations. Within each of these groups, we examine the effects of zinc treatment in severely-moderately under-nourished and well-nourished children. To define nutritional status in this population not clinically identified as having severe acute malnutrition, we derive weight for age z scores using WHO reference population data [13]. Children with weight for age z-scores<-2 are classified retrospectively as severely-moderately under-nourished, while those with z-scores≥-2 are considered well-nourished [13].
Handling of missing data
As there were missing data in the variables (listed in Table 1) we use for analysis (see Table S1 in Online Supplementary Document for data completeness (%)), we assume these data were missing at random (MAR) and to maintain the effective sample size, multiple imputation methods are used to fill in the missing data [14]. In this approach, we impute 10 data sets [15] using chained equations which allow for the correct specification of the distribution of each variable. We then examine the plausibility of imputed values by comparing the distribution of observed values with that of imputed values (see Figure S1 in Online Supplementary Document).
Group 1 (1-5 months) | Group 2 (6-59 months) | |||||||||||
---|---|---|---|---|---|---|---|---|---|---|---|---|
Variable | Before PS weighting | After PS weighting | Before PS weighting | After PS weighting | ||||||||
Zinc (n = 1181) | No zinc (n = 464) | ASMD | Zinc | No zinc | ASMD | Zinc (n = 8853) | No zinc (n = 2693) | ASMD | Zinc | No zinc | ASMD | |
Discrete variables | ||||||||||||
Pulse: | 0.04 | 0.06 | 0.08 | 0.03 | ||||||||
Normal | 93.9 | 93.7 | 93.9 | 92.9 | 94.6 | 92.7 | 94.6 | 93.8 | ||||
Weak | 6.1 | 6.3 | 6.1 | 7.1 | 5.4 | 7.3 | 5.4 | 6.2 | ||||
AVPU: | 0.07 | 0.09 | 0.06 | 0.00 | ||||||||
Alert | 97.8 | 96.6 | 97.8 | 97.4 | 97.7 | 97.0 | 97.7 | 97.7 | ||||
Verbal response | 2.2 | 3.4 | 2.2 | 2.6 | 2.3 | 3.0 | 2.3 | 2.3 | ||||
Capillary refill: | 0.12 | 0.03 | 0.03 | 0.00 | ||||||||
≤3 seconds | 95.6 | 96.3 | 95.6 | 95.0 | 95.3 | 94.4 | 95.3 | 95.3 | ||||
>3 seconds | 0.7 | 1.5 | 0.7 | 0.8 | 1.1 | 1.4 | 1.1 | 1.1 | ||||
Intermediate | 3.7 | 2.2 | 3.7 | 4.2 | 3.6 | 4.2 | 3.6 | 3.6 | ||||
Sunken eyes: | 0.18 | 0.06 | 0.15 | 0.01 | ||||||||
Yes | 29.0 | 20.6 | 29.0 | 26.3 | 33.6 | 26.8 | 33.6 | 32.6 | ||||
No | 71.0 | 79.4 | 71.0 | 73.7 | 66.4 | 73.2 | 66.4 | 67.4 | ||||
Skin pinch: | 0.03 | 0.07 | 0.05 | 0.02 | ||||||||
1–2 seconds | 23.0 | 22.4 | 23.0 | 20.1 | 22.9 | 21.4 | 22.9 | 22.6 | ||||
2> seconds | 67.7 | 68.0 | 67.7 | 72.1 | 8.1 | 9.2 | 8.1 | 8.6 | ||||
Immediate | 9.3 | 9.6 | 9.3 | 7.8 | 69.0 | 69.4 | 69.0 | 68.8 | ||||
Blood transfusion order: | 0.01 | 0.05 | 0.10 | 0.02 | ||||||||
Yes | 2.9 | 2.8 | 2.9 | 2.5 | 2.4 | 4.0 | 2.4 | 2.7 | ||||
No | 97.1 | 97.2 | 97.1 | 97.5 | 97.6 | 96.0 | 97.6 | 97.3 | ||||
Able to drink: | 0.14 | 0.06 | 0.06 | 0.00 | ||||||||
Yes | 84.4 | 78.7 | 84.4 | 82.2 | 84.7 | 82.6 | 84.7 | 84.7 | ||||
No | 15.6 | 21.3 | 15.6 | 17.8 | 15.3 | 17.4 | 15.3 | 15.3 | ||||
Skin temperature: | 0.11 | 0.06 | 0.05 | 0.02 | ||||||||
Elbow | 3.0 | 4.1 | 3.0 | 4.0 | 3.6 | 4.0 | 3.6 | 3.8 | ||||
Hand | 95.4 | 92.9 | 95.4 | 94.5 | 94.3 | 93.4 | 94.2 | 94.0 | ||||
Shoulder | 1.6 | 3.0 | 1.6 | 1.5 | 2.1 | 2.6 | 2.2 | 2.2 | ||||
Child sex: | 0.01 | 0.03 | 0.05 | 0.01 | ||||||||
Male | 55.2 | 54.6 | 55.2 | 54.3 | 55.2 | 53.1 | 55.2 | 55.8 | ||||
Female | 44.8 | 45.4 | 44.8 | 45.7 | 44.8 | 46.9 | 44.8 | 44.2 | ||||
Pallor: | 0.12 | 0.06 | 0.14 | 0.02 | ||||||||
None | 87.8 | 85.8 | 87.8 | 86.9 | 88.0 | 83.4 | 88.0 | 87.6 | ||||
Mild/moderate | 9.2 | 12.3 | 9.2 | 9.9 | 9.5 | 12.3 | 9.5 | 9.8 | ||||
Severe | 3.0 | 1.9 | 3.0 | 3.1 | 2.5 | 4.3 | 2.5 | 2.7 | ||||
Fever: | 0.07 | 0.07 | 0.01 | 0.05 | ||||||||
Yes | 81.3 | 78.7 | 81.3 | 78.7 | 75.5 | 75.9 | 75.5 | 11.4 | ||||
No | 18.7 | 20.3 | 18.7 | 20.3 | 24.5 | 24.1 | 24.5 | 89.6 | ||||
Convulsions: | 0.05 | 0.04 | 0.08 | 0.01 | ||||||||
Yes | 7.5 | 8.6 | 7.5 | 7.9 | 11.2 | 13.2 | 11.2 | 11.4 | ||||
No | 92.5 | 91.4 | 92.5 | 92.1 | 88.8 | 86.8 | 88.8 | 88.6 | ||||
Vomiting: | 0.05 | 0.10 | 0.17 | 0.01 | ||||||||
Yes | 60.6 | 58.1 | 60.6 | 55.6 | 81.3 | 74.7 | 81.3 | 80.9 | ||||
No | 39.4 | 41.9 | 39.4 | 44.4 | 18.7 | 25.3 | 18.7 | 19.1 | ||||
Hospital referral: | 0.24 | 0.01 | 0.17 | 0.01 | ||||||||
Yes | 17.2 | 27.1 | 17.2 | 17.0 | 16.4 | 21.8 | 16.4 | 16.9 | ||||
No | 82.8 | 72.9 | 82.8 | 83.0 | 83.6 | 78.2 | 83.6 | 83.1 | ||||
Severe wasting: | 0.19 | 0.04 | 0.18 | 0.01 | ||||||||
Yes | 5.6 | 11.2 | 5.6 | 5.2 | 5.7 | 10.4 | 5.7 | 5.9 | ||||
No | 94.4 | 88.8 | 94.4 | 94.8 | 94.3 | 89.6 | 94.3 | 94.1 | ||||
Thrush: | 0.03 | 0.01 | 0.08 | 0.01 | ||||||||
Yes | 3.9 | 4.3 | 3.9 | 4.0 | 3.4 | 4.9 | 3.4 | 3.1 | ||||
No | 96.1 | 95.7 | 96.1 | 96.0 | 96.6 | 95.1 | 96.6 | 96.9 | ||||
Oedema: | 0.09 | 0.10 | 0.05 | 0.01 | ||||||||
Face | 0.2 | 0.0 | 0.2 | 0.0 | 0.3 | 0.4 | 0.3 | 0.4 | ||||
Foot | 1.1 | 1.0 | 1.1 | 1.5 | 1.3 | 1.8 | 1.3 | 1.4 | ||||
Knee | 0.2 | 0.2 | 0.2 | 0.2 | 0.1 | 0.2 | 0.1 | 0.1 | ||||
None | 98.5 | 98.8 | 98.5 | 98.3 | 98.3 | 97.6 | 98.3 | 98.1 | ||||
Oral fluid: | 0.26 | 0.03 | 0.32 | 0.00 | ||||||||
Administered | 83.8 | 75.1 | 83.8 | 83.7 | 87.9 | 75.2 | 87.9 | 88.0 | ||||
Not administered | 16.2 | 24.9 | 16.2 | 16.3 | 12.1 | 24.8 | 12.1 | 12.0 | ||||
IV fluid: | 0.02 | 0.07 | 0.07 | 0.00 | ||||||||
Administered | 35.5 | 36.8 | 35.5 | 37.4 | 33.7 | 37.9 | 33.7 | 33.8 | ||||
Not administered | 64.5 | 63.2 | 64.5 | 62.6 | 66.3 | 62.1 | 66.3 | 66.2 | ||||
Wheeze: | 0.16 | 0.01 | 0.10 | 0.00 | ||||||||
Present | 3.7 | 7.8 | 3.7 | 3.6 | 2.1 | 3.4 | 2.1 | 2.1 | ||||
Absent | 96.3 | 92.2 | 96.3 | 96.4 | 97.9 | 96.6 | 97.9 | 97.9 | ||||
Hospital: | 0.74 | 0.13 | 0.59 | 0.05 | ||||||||
H 1 | 10.2 | 2.4 | 10.2 | 7.4 | 7.9 | 4.1 | 7.9 | 7.5 | ||||
H 2 | 8.6 | 8.6 | 8.6 | 8.3 | 9.2 | 9.1 | 9.2 | 9.4 | ||||
H 3 | 10.8 | 8.0 | 10.8 | 12.2 | 9.5 | 10.8 | 9.5 | 9.3 | ||||
H 4 | 5.3 | 4.5 | 5.3 | 6.1 | 6.9 | 6.7 | 6.9 | 6.7 | ||||
H 5 | 4.8 | 3.9 | 4.8 | 4.2 | 5.6 | 5.8 | 5.6 | 5.8 | ||||
H 6 | 11.9 | 5.2 | 11.9 | 11.3 | 12.1 | 6.8 | 12.1 | 11.7 | ||||
H 7 | 6.5 | 4.3 | 6.5 | 6.2 | 7.1 | 5.4 | 7.1 | 7.2 | ||||
H 8 | 11.9 | 9.7 | 11.9 | 12.3 | 14.6 | 9.5 | 14.6 | 15.6 | ||||
H 9 | 7.3 | 7.5 | 7.3 | 7.7 | 6.0 | 4.2 | 6.0 | 5.7 | ||||
H 10 | 11.3 | 15.3 | 11.3 | 12.1 | 7.7 | 9.6 | 7.7 | 7.9 | ||||
H 11 | 3.7 | 20.5 | 3.7 | 3.8 | 2.6 | 15.6 | 2.6 | 2.7 | ||||
H 12 | 0.5 | 0.0 | 0.5 | 4.6 | 0.5 | 1.3 | 0.5 | 0.5 | ||||
H 13 | 3.9 | 8.6 | 3.9 | 2.6 | 5.9 | 8.4 | 5.9 | 6.0 | ||||
H 14 | 3.3 | 1.4 | 3.3 | 1.2 | 4.4 | 2.7 | 4.4 | 4.0 | ||||
Diarrhoea >14 days: | 0.03 | 0.02 | 0.04 | 0.01 | ||||||||
Yes | 3.0 | 1.9 | 3.0 | 2.6 | 2.9 | 3.9 | 2.9 | 2.7 | ||||
No | 97.0 | 98.1 | 97.0 | 97.4 | 97.1 | 96.1 | 97.1 | 97.3 | ||||
HIV: | 0.07 | 0.02 | 0.06 | 0.01 | ||||||||
Positive | 1.3 | 2.2 | 1.3 | 1.3 | 1.4 | 2.4 | 1.4 | 1.5 | ||||
Negative | 98.7 | 97.8 | 98.7 | 98.7 | 98.6 | 97.6 | 98.6 | 98.5 | ||||
Pneumonia: | 0.19 | 0.01 | 0.19 | 0.02 | ||||||||
Positive | 49.4 | 58.7 | 49.4 | 49.8 | 28.4 | 36.1 | 28.4 | 27.6 | ||||
Negative | 50.6 | 41.3 | 50.6 | 50.2 | 71.6 | 63.9 | 71.6 | 72.4 | ||||
Malaria: | 0.22 | 0.00 | 0.09 | 0.01 | ||||||||
Positive | 23.1 | 13.5 | 23.1 | 23.0 | 29.0 | 24.9 | 29.0 | 28.7 | ||||
Negative | 76.9 | 86.5 | 76.9 | 77.0 | 71.0 | 75.1 | 71.0 | 71.3 | ||||
Meningitis: | 0.18 | 0.01 | 0.09 | 0.01 | ||||||||
Positive | 4.1 | 8.4 | 4.1 | 4.5 | 4.7 | 6.8 | 4.7 | 4.8 | ||||
Negative | 95.9 | 91.6 | 95.9 | 95.5 | 95.3 | 93.2 | 95.3 | 95.2 | ||||
Continuous variables | ||||||||||||
Weight – kg (mean) | 5.8 | 5.6 | 0.13 | 5.8 | 5.8 | 0.00 | 10.2 | 10.8 | 0.04 | 10.2 | 10.2 | 0.01 |
Temperature (mean) | 37.7 | 37.9 | 0.20 | 37.7 | 37.7 | 0.02 | 37.7 | 37.7 | 0.06 | 37.7 | 37.7 | 0.01 |
Length of illness – days (mean) | 5.4 | 5.2 | 0.01 | 5.4 | 5.4 | 0.05 | 4.8 | 5.9 | 0.10 | 4.8 | 4.9 | 0.01 |
PS – propensity score, ASMD – absolute standardised mean difference, AVPU – alert, verbal, pain, unresponsive
*Summary of discrete variables are presented as percentages while continuous variables are presented as means.
The plausibility of MAR assumption is examined by conducting sensitivity analyses under missingness not at random (MNAR) assumption [16]. Under MAR, we assume the clinicians unintentionally failed to record such clinical data or fill some sections of the treatment sheets and the missingness process is therefore ignorable thus the recorded data by clinicians would plausibly be predictive of missing data [16]. However, it is also possible that data are missing for reasons that are not random but linked to the data recording process. These reasons are not necessarily known but could create a scenario in which data are MNAR. See the supplementary material for the MNAR patterns considered.
Handling non-random allocation of zinc supplement
As CIN comprises data from routine care settings, not being prescribed zinc cannot be considered random. In fact, this would be as a result of failure to adhere to national guidelines. Analyses must try and account for any potential bias in treatment allocation. To help create balance in population characteristics amongst those with and without zinc prescription, we compare propensity score full-matching and weighting [17,18]. A propensity score describes the probability of a patient being prescribed zinc based on measured characteristics, in this case clinical signs, symptoms, co-treatments and comorbidities [18]. Full-matching and weighting adjustments are done for each of the 10 imputed data sets and absolute standardised mean differences (ASMD) used as diagnostic checks for covariate balance and overlap [19,20] between zinc and non-zinc groups. We then base outcome analysis on the best performing method resulting in minimised ASMD for most of the variables. Covariables used in creating propensity scores in all the analyses include key signs and symptoms suggested for diagnosing and assessing severity of diarrhoea and dehydration in the Kenyan paediatric guidelines together with variables considered a priori to influence the clinical outcomes of interest such as fluid regimen prescribed and comorbidities (see Table S1 in Online Supplementary Document for a summary of these variables, and sub section (d) of Online Supplementary Document for the process of fitting the propensity score models).
Modelling of time to being discharged alive
We consider time to being discharged alive as the primary outcome. Mortality is treated as a competing risk as it would preclude the chance of a patient being discharged alive. To allow for covariates with varying effects across the discharge time points, we use a flexible modelling approach suggested by Scheike (2011) [21]. Both Kolmogorov-Smirnov and Cramer von Mises test statistics [21] are used to determine whether covariates have varying or constant effects across time points prior to discharge. Patients who absconded, were referred to other hospitals and those who had length of stay greater than 15 days (as zinc should be administered for two weeks and should influence the outcome of acute diarrhoea) are censored.
For each of the imputed and then propensity score adjusted data sets, Scheike’s flexible regression models are fitted while adjusting for all the variables (see Table S1 in Online Supplementary Document) also used in the corresponding propensity score models. Only adjusted treatment effectiveness estimates are pooled across the imputed data sets using Rubin rules [22] to obtain a single estimate. This analysis also reports estimates without propensity score adjustments. An additive interaction [23] is used in modelling the use of zinc by nourishment status. As exploratory analyses, time to experiencing inpatient mortality is modelled on the same propensity score adjusted data sets.
Sensitivity analysis using an instrumental variable
As the CIN data are limited to the recorded variables, we use timing of admission [24,25] as an instrumental variable to examine the potential influence of any unmeasured variables in all the analysis of time to being discharged alive [24]. Multiple studies including Berkley (2004), Bell (2001), Freemantle (2015), Meacock (2016) and Aldridge (2016) have demonstrated that patients who were admitted during the weekend experienced worse outcomes compared to those admitted during the weekdays [26-30] – which may be an indication of poor quality of care and treatment during the weekend. This, in theory, implies that the type of treatment and care received depend on the day of admission – and which later determines the type of health outcome of the patient. See the supplementary material for the process followed in fitting the instrumental variable models.
Inclusion and exclusion
A total of 1645 and 11 546 children were eligible for analysis in groups 1 (aged 1-5 months) and 2 (aged 6-59 months) respectively. About 72% (1-5 months) and 77% (6-59 months) were prescribed zinc supplements (Figure 1). See detailed distribution of patients by clinical signs, cotreatments, comorbidities and hospital in Table 1.
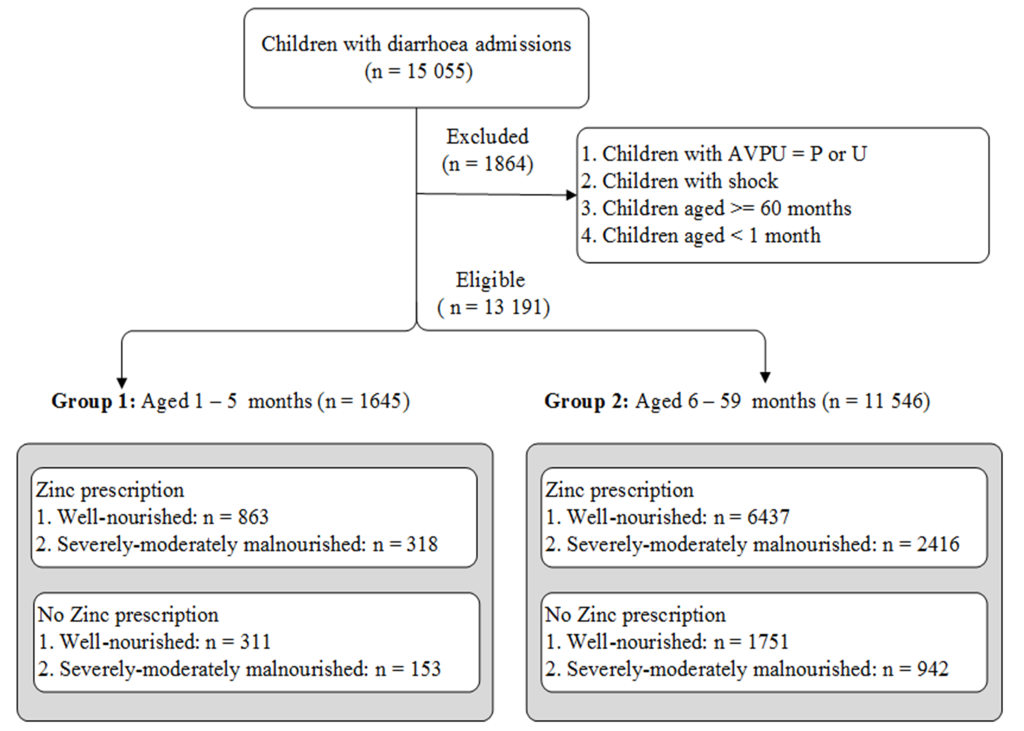
Outcome analysis
Exploring probability of being discharged alive in groups 1 and 2
Cumulative incidence functions were explored in the raw data sets to describe the probability of being discharged alive over time (Figure 2). Approximately 60% of the children were discharged by the fifth day of their stay in the hospital. Mortality was approximately 9% (zinc = 82/1181, non-zinc = 64/464) and 5% (zinc = 353/8853, non-zinc = 226/2693) in age groups 1 and 2 respectively. Approximately 2.6% (zinc = 25/1181, non-zinc = 18/464) and 1.8% (zinc = 119/8853, non-zinc = 84/2693) in groups 1 and 2 were censored as they either absconded, were referred to other hospitals and or had length of stay greater than 15 days. In both age groups (and without propensity score adjustment) children who were prescribed zinc were discharged sooner than those who were not prescribed zinc. However, this difference was not clear for those who were discharged within three days of hospitalisation.
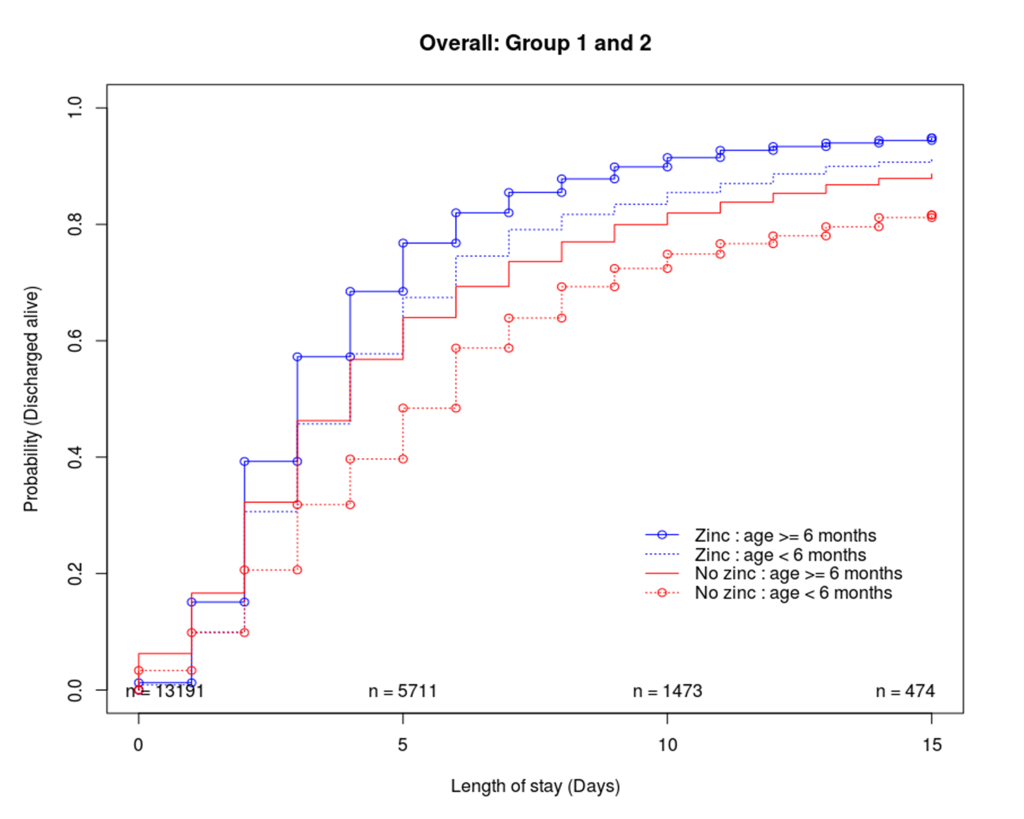
Modelling time to discharge
For group 1, the performance of optimal full matching was comparable to that of weighting, while weighting performed better than optimal full matching in group 2 (see Table 1, Figures S2 and S3 in Online Supplementary Document). Thus modelling time to discharge analyses for both age groups were based on propensity score weighted data sets. In propensity score weighted data sets, all the variables had ASMD <10% (apart from hospital variable in group 1) – which indicated the covariate imbalance between the zinc and non-zinc groups were minimised. The estimated sub-distribution hazard ratios (SHR) in multivariable PS weighted models (of being discharged alive in the zinc group vs the non-zinc group) were 1.25 (95% CI = 1.07, 1.46) and 1.17 (95% CI = 1.10, 1.24) in age groups 1 and 2 respectively. In a pooled analysis (across the two age groups), the overall effectiveness of zinc was 1.17 (95% CI = 1.11, 1.24). This can be interpreted as a child being prescribed zinc having on average a 17% higher chance of being discharged at any point in time than a child not prescribed zinc (Figure 3). Multivariable models without propensity score weighting produced somewhat higher effect estimates (SHR 1.33 (95% CI = 1.16, 1.53) and 1.30 (95% CI = 1.23, 1.38) for age groups 1 and 2, respectively) suggesting propensity score weighting may be adjusting for some potential confounding favouring zinc prescription.
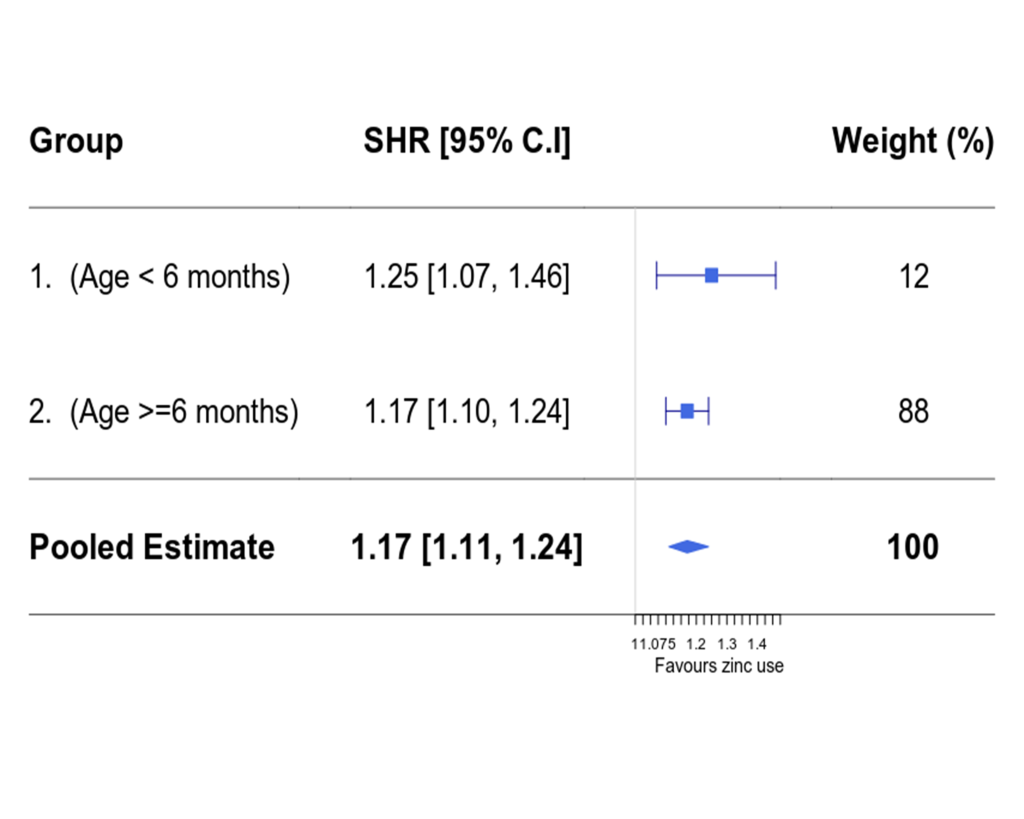
Zinc effectiveness for well-nourished and under-nourished children
Approximately a third of children in both age groups were severely-moderately under-nourished (group 1 = 28.6% and group 2 = 29.1%). The additive interaction resulted in four sub-groups of children including those who were: (a) well-nourished with zinc prescribed; (b) well-nourished without zinc prescribed; (c) under-nourished with zinc prescribed and; (d) under-nourished without zinc prescribed. Patient characteristics were approximately similar across the four sub-groups in both age groups (see Tables S2 and S3 in Online Supplementary Document). The propensity score adjusted Scheike’s flexible model estimates (in both age groups) suggested that those who were prescribed zinc and were well-nourished were more likely to be discharged sooner, followed by those who were not prescribed zinc and were well-nourished, then those who were prescribed zinc but were under-nourished and lastly those who were not prescribed zinc but were under-nourished (Table 2). Results interpreted in a similar way were found using propensity score unadjusted data (see Table S4 in Online Supplementary Document).
1-5 mo | 6-59 mo | |
---|---|---|
Zinc-undernourished* | 0.82 (0.67, 1.01) | 0.85 (0.79, 0.91) |
No zinc-wellnourished* | 0.88 (0.74, 1.04) | 0.90 (0.84, 0.98) |
No zinc-undernourished* | 0.63 (0.49, 0.82) | 0.64 (0.58, 0.70) |
Zinc-wellnourished (reference group)* | - | - |
SHR – subdistribution hazard ratio
*Zinc-undernourished – received zinc but were under-nourished, No zinc-wellnourished – were wellnourished but did not receive zinc, No zinc-undernourished – were undernourished but did not receive zinc, Zinc-wellnourished – received zinc and were well nourished.
Sensitivity analysis using an instrumental variable
The estimated SHRs (comparing zinc vs no zinc) using the instrumental variable for children aged 1-5 and 6-59 months were 1.24 (95% CI = 1.18, 1.30) and 1.31 (95% CI = 1.27, 1.35) respectively – and these were consistent with those obtained in Figure 3.
Modelling time to experiencing inpatient mortality (exploratory analysis)
Both Kolmogorov-Smirnov and Cramer Von Mises tests showed that the use of zinc had varying effects on mortality (across the discharge time points) both for children aged 1-5 and 6-59 months (see Table S5 in Online Supplementary Document). Thus, time specific estimates (with corresponding 95% C.I) were modelled using Scheike’s regression and the results presented in Figure 4 (Panel A and Panel B). For children aged 1-5 months, zinc seemed to have no effect on mortality for those who stayed in the hospital for about a week. However, beyond one week, those who were prescribed zinc seemed to have significantly reduced risk of dying. While zinc use was associated with reduced mortality for children aged 6-59 months across all the discharge time points.
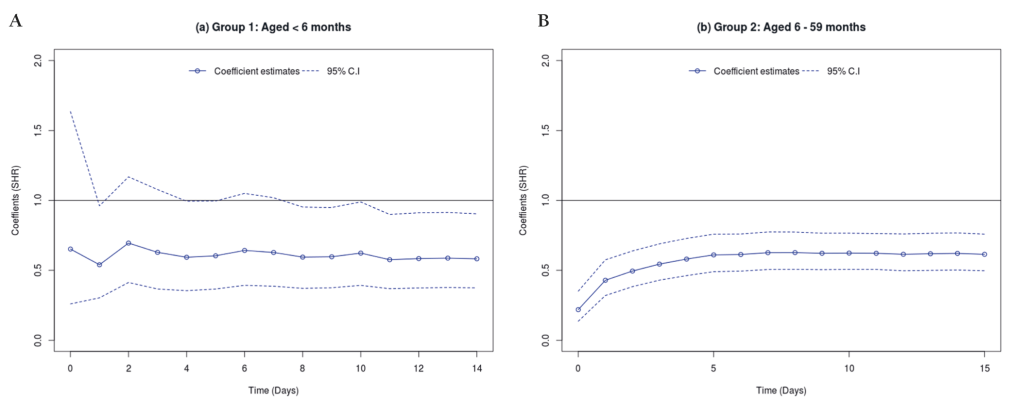
Analysis under missingness not at random
The Scheike’s model zinc treatment effectiveness estimates, under MNAR, were as presented in Figures S4, Panel A and B, in Online Supplementary Document. The pattern specific trends were in opposite directions although in group 2 the effect estimate was larger where there was most missingness. However, the pooled effects were consistent with those observed in Figure 3. This indicates that the earlier assumption of data missing at random was plausible.
The results indicate that zinc may be beneficial in reducing time to discharge for children aged 1–59 months admitted with diarrhoea. This may be generalizable to children who have fever, normal pulse, are alert, vomiting, have capillary refill ≤3 minutes, those who do not require blood transfusion, and have no pallor, convulsions, thrush or oedema. An association with benefit is seen for both well-nourished and severely – moderately under-nourished children. This analysis supports and strengthens the current treatment policy used in Kenya which was implemented on the basis of limited evidence supporting the use of zinc in those aged less than 6 months and in well-nourished children. Two previous trials conducted in Africa (Ethiopia, n = 177, and Nigeria, n = 60) with children under 6 months [5,7] showed no effect of zinc in reducing diarrhoea duration. Our analyses offer the advantage of larger sample size but have the limitation of being based on a non-randomised study. Previous studies [7] have reported no serious adverse effects associated with the use of zinc apart from a risk of increased vomiting (within 10 minutes of administration). We were unable to examine differences in this possible adverse effect but if it occurs it does not seem to be increasing the length of stay for hospitalised children with diarrhoea. The findings therefore seem to contribute additional evidence on the likely value of zinc in support of its routine use for hospitalised children with diarrhoea in Kenya.
Strengths and limitations
There are limitations whenever one uses routine, observational data sets. The WHO recommends two measures of malnutrition – m id-upper arm circumference and weight for height – but we were not able to use these as they are poorly documented in hospitals. Instead we used weight for age z score, associated with undernourishment, as a proxy. Data on specific covariables were also sometimes missing. We used multiple imputation to overcome this challenge assuming data were MAR. Methodologically, this assumption seemed plausible as estimates of the effects associated with zinc under assumptions of MAR and MNAR were approximately similar.
Comparative effectiveness analyses using observational data sets offer the potential advantage of evaluating “real world” effectiveness of treatments. In part, this is because children who may not qualify to be part of RCTs may be included in these observational studies. The use of propensity scores and multiple imputation provided a means to analytically handle the challenges of non-random allocation and missing data by creating samples of patients that are comparable in terms of observed signs, symptoms, co-treatments and comorbidities. Analyses of these samples provided biologically plausible results showing the benefits of zinc in reducing time to discharge for all children below five years. However, as analytic methods used in observational studies may not completely eliminate unobservable bias [31,32], we conducted sensitivity and exploratory analyses with the use of an instrumental variable and time to experiencing mortality respectively. The findings of instrumental variable analyses were consistent with those obtained with the propensity score methods. Also, our analysis demonstrated that zinc prescription was associated with reduced mortality – and this was consistent with the findings of a community trial in Bangladesh, which showed reduced chances of mortality by 50% [33]. These findings on mortality, however, are in contrast with those of RCTs included in the systematic reviews [7,34], which showed no effect of zinc on mortality. It may therefore be that residual bias is influencing our reported findings. It is plausible that zinc, an oral medication, is not prescribed for children who are thought to be more severely ill by clinicians based on their gut feeling rather than their recorded clinical features. Gut feeling has been identified as an important predictor of severe illness in some studies [35]. The possibility of this form of allocation bias is perhaps supported by the finding, in children 6-59 months, that zinc is associated with reduced mortality from the first day of admission as biologically zinc may not be expected to have such a rapid protective effect on this outcome.
Our finding that zinc is associated with shorter time to discharge is consistent with meta-analyses of RCTs but extends this observation to less studied populations. RCTs have, however, not shown an advantageous effect of zinc on mortality in acute diarrhoea. The protective association of zinc against mortality we see does raise the possibility that there is residual (unobservable) bias in our observational analyses possibly due to clinicians allocating this treatment to less sick children. Despite this caveat, and as zinc is well tolerated and cheap, our results support the continued use of routine zinc supplementation in all children aged 1-59 months hospitalised with diarrhoea in Kenya and probably elsewhere in Africa.