Many low- and middle-income countries (LMICs) are characterised by high levels of socioeconomic inequality and uneven access to clinical and preventive services, leading to unacceptably wide differences in health outcomes between rich and poor [1]. In many cases this is exacerbated by weak systems of governance, which hinder the efficient and fair distribution of resources. Tackling such inequalities is one of the Sustainable Development Goals proposed by the United Nations (SDG #10), and is echoed in the World Health Organization’s (WHO) campaign to promote Universal Health Coverage as a fundamental human right [2].
While the association between socioeconomic status (SES) and health has been relatively well-studied in high income countries, much less is known about how these factors interact in LMICs or how changing local contexts influence patterns of health inequality in these settings. Obtaining a better understanding of this is vital for informing effective, targeted, responsive, and equitable health and social services [3].
LMICs today are struggling to reconcile growing population health needs with finite resources but these challenges are magnified in those facing the greatest economic and workforce constraints. Interest in making better use of data and information systems to improve the effectiveness, efficiency and safety of health care is growing worldwide. Within the global health and development community this has led to a recognition of the value of large, digital data sets for Health Systems Strengthening, with better capture, storage, exchange and use of data seen as a means of improving the efficiency, transparency and accountability of health care processes [4]. Making better use of “big” data also offers opportunities for research and public health analytics, to better understand the frequency, severity, causes, correlates and consequences of injury and illness, including health inequalities [5]. The release of WHO’s new, “fully electronic” International Classification of Diseases scheme in June 2018 marks an acknowledgement of this need for better data [6].
Access to reliable and meaningful data can help responsible policymakers and health leaders to make informed choices about geographic areas or population subgroups with the greatest need, and thus to design appropriately targeted interventions to reduce inequalities. Good data also provide a means of evaluating the effectiveness of such interventions once implemented. Unfortunately, in many LMICs, data appropriate for monitoring health inequalities are either not collected, hard to find or too unreliable to use, creating a knowledge vacuum that can be hard to fill with statistical extrapolation. Many sub-Saharan African countries lack accurate and complete Vital Statistics (birth and death registration) or reliable census-based population health estimates [7]. In addition, information from service facilities is often inadequate, either because of differences in local case-mix or because facilities lack the tools or training to be able to accurately record patient data (eg, demographics, diagnoses, interventions, outcomes) [3,7].
To overcome gaps in national data sets, many LMICs have come to rely on internationally funded Demographic and Health Surveys (DHS) to estimate population health status and its inequalities. These well-designed, internationally coordinated surveys collect household-level SES variables and self-reported demographic and health outcomes, through standardized questionnaires. However, current DHS have three inherent limitations when it comes to monitoring health inequalities:
-
The vast effort and expense involved in national door-to-door surveys mean that these rarely take place more than twice per decade. This is insufficient for monitoring variations in health status and inequalities which can change rapidly in LMICs, eg, water- or vector-borne diseases after floods; malnutrition after drought or pest-related crop failure; warfare; or sudden refugee influx.
-
The population captured by DHS is often too small, in statistical terms, to estimate health outcomes below the level of regions. However in LMICs the planning and management of healthcare, social care and other government services are steadily being devolved to sub-regional districts and municipalities. In their current form, DHS are unable to meet the data needs of those key local jurisdictions.
-
DHS are expensive and technically demanding, which has led to LMICs becoming dependent on international donors and foreign experts for their funding design, analysis and reporting. With many high income countries now re-thinking their international aid commitments, LMICs face increasing pressure to develop ‘home-grown’ alternatives.
Developing sustainable, national strategies for monitoring health inequalities ideally requires data capture at the local level [3], which can then be aggregated to provide regional and national data. It also requires innovative uses of existing data sources, including public sector administrative data, business data and new forms of data emerging from society’s use of Information and Communications Technology (ICT).
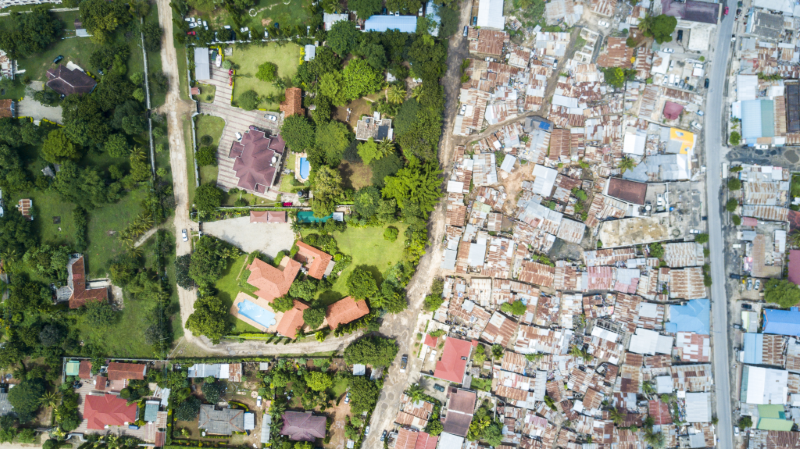
Tanzania provides an illustrative example of the challenges involved in estimating health inequalities in the context of LMICs, as well as opportunities for improvement using existing and emerging data sources.
Tanzania is a relatively stable but nonetheless low-income African nation with a per capita GDP of USD 879 in 2016 and an economy heavily dependent on small-scale farming. Annual per capita health expenditure is approximately 166 USD, around 20% via government-funded health care services, 20% from private insurance (eg, occupational), 20% from out-of-pocket spending, and 40% from international aid that directly supports health programmes or facilities [8].
Two key sources of health and SES data in the past
Tanzania has participated in health data collection through DHS since 1991-92. Tanzania’s most recent DHS report (2015-16) explicitly documents inequalities in a dozen fertility and health outcome indicators, across levels of household education or assets (wealth quintile) [9].
Tanzania’s 2015-16 DHS included interviews with nearly 20 000 adults. However, with a national population now over 50 million, this can, at best, provide reliable estimates of most health indicators only to the level of Regions, of which Tanzania (including Zanzibar) has thirty. For less common outcomes, such as mid-life and maternal deaths, and for judging health inequalities across SES strata, the latest Tanzanian DHS can provide reliable estimates only down to the level of Zones (clusters of Regions) [9]. Yet the current National Health Plan aims to decentralize decision-making to Regional level and eventually to District/Municipality level [10].
In parallel with DHS, research-oriented Demographic and Health Surveillance Systems (DHSS) have been established in some areas, providing “gold-standard” demographic and health data at the household level [7]. The Ifakara Health Institute (IHI), where two of the authors are based, established DHSSs in the mid-1990s to measure local health indicators for the Tanzania Essential Health Intervention Project (TEHIP) [11]. It has since continued to operate DHSS in small-urban ones (Ifakara) and rural Districts (Kilombero, Ulanga, and Rufiji). Workers skilled in ‘verbal autopsy’ visit regularly enumerated homes to collect information about medical causes of illness and death (abstracted from narrative accounts) as well as demographics and household wealth, based on validated tools for assessing SES in agricultural subsistence economies [7], These DHSS generate high-quality data on household-level SES, demographics and health outcomes, which can be merged with outcomes data from health facilities to better estimate local-area health inequalities at the sub-District/Ward level (populations typically 5000-20 000). Because of their granularity and geographic precision, DHSS data provide a valuable benchmark against which to evaluate the validity of new data-sources for measuring local-area inequalities, as described below.
Harnessing new forms of data for estimating local-area average SES
The Tanzanian Government has recently set out its intentions to build upon and augment information systems and infrastructure to obtain “better data for better health”, in its new Digital Health Investment Roadmap [12], whilst recognising the challenges of converting from paper-based systems. Against this backdrop, other digital innovations also offer opportunities to improve health monitoring, whilst presenting unique potential for understanding health inequalities.
Mobile phones had reached 72% of the population of Tanzania by 2017, according to recent statistics [13]. This market is dominated by five regulated companies who maintain detailed records on customers and transactions, providing a potentially rich source of data indicative of SES at a micro-local level. Amongst the most promising such indicators are per capita/household subscription costs, total purchased call-time, density of licensed service suppliers, and tax revenues collected from licenses and purchases.
Social media, such as Facebook, WhatsApp and Twitter, are also widely accessible in Tanzania, with around 5M users having mobile access [13]. Much of the information posted by citizens on these platforms is geotagged, presenting potential opportunities to harvest anonymised local indicators of economic activity, demographics and health status, along with sentiments about social, financial and health inequalities. Similarly, just as Internet search analytics are being deployed for infectious disease surveillance, inferences about disposable income and health needs may be inferred from consumer search data. Private companies in Tanzania are already using such data to obtain insights about consumer behaviour, spending power or social attitudes, and the government is also a keen user of these insights, although not without controversy.
Mobile banking services are also widespread in Tanzania and other LMICs, substituting for the lack of physical banking options for many citizens [14]. Anonymised administrative data from these services can provide information on local-area financial resources and transactions, which may be invisible through official channels in cash-based LMICs economies. Indeed, India has recently transformed its currency to a largely digitised and thus observable one, at the same time providing a virtual identity for every citizen [http://cashlessindia.gov.in]
Converging these digital data sources with conventionally collected health outcomes could help to improve the monitoring of health inequalities, and provide cost-effective options for scaled and sustainable reporting.
Obtaining buy-in from relevant stakeholders is essential for public health surveillance based on data reuse. For a start, data holders, such as Government Departments, telecommunications and social media companies, as well as local and regional health providers, need to be prepared to share their anonymized data, which may be protected under their information governance policies, politically or organisationally sensitive, or commercially valuable. Citizens may also feel uncomfortable with the use of such data for purposes other than originally intended; for example, they may be concerned about the disclosure of potentially stigmatising health conditions or untaxed income. The risk of data breaches or political misuse of social media postings illustrates the dilemma between harnessing data for public benefit and damaging public trust [15]. For this reason engaging and involving citizens and other stakeholders will be essential if such systems are to be acceptable.
The quality of existing data can vary widely across sources, creating challenges for the use of these data for any new or original purpose. Socio-technical factors are also relevant here, such as whether organisations regard the capture and maintenance of clean data as a priority, and invest in a trained workforce to collect and process such data, as are technical barriers to the extraction and linkage of heterogeneous data types. Developing innovative health inequalities monitoring is further complicated by the need to capture and integrate information from multiple sectors and “data cultures”.
Understanding decision-makers’ views about potential home-grown methods of health inequalities assessment will also be vital for assessing their potential to drive interventions for reducing health inequalities. Data alone are unlikely to help unless they are accompanied by effective action cycles, and the will to implement them [4].
Even with the best available data, making effective use of such data also depends on having the statistical and epidemiological capacity to do so, and for this reason, investment in training and retention of specialists is also essential.
We are currently consulting with Tanzanian stakeholders to explore the feasibility and acceptability of augmenting existing DHS data with these novel data sources, as a means of cost-effectively generating geographically fine-grained and timely data on health inequalities, based on measures of local-area average SES. This is an essential first step towards improving Tanzania’s and other LMICs’ ability to efficiently and independently monitor and act upon health inequalities. Looking forward, there is also a need to progress this discussion beyond improving data on health inequalities to purposefully using it to understand their complex causes, consequences and possible solutions. As SDG#10 implies, such action is critical for achieving a more equitably developed world.